LAB 07.01 - Optimization for ML
Contents
LAB 07.01 - Optimization for ML¶
!wget --no-cache -O init.py -q https://raw.githubusercontent.com/rramosp/ai4eng.v1/main/content/init.py
import init; init.init(force_download=False); init.get_weblink()
from local.lib.rlxmoocapi import submit, session
session.LoginSequence(endpoint=init.endpoint, course_id=init.course_id, lab_id="L07.01", varname="student");
from sklearn.datasets import make_moons
import numpy as np
import matplotlib.pyplot as plt
import pandas as pd
from local.lib import mlutils
%matplotlib inline
Dataset¶
you have data in a matrix \(\mathbf{X} \in \mathbb{R}^{m\times n}\) and the corresponding labels \(\mathbf{y} \in \mathbb{R}^m\).
each row in matrix \(\mathbf{X}\) contains one data point \(\mathbf{x}^{(i)}\)
and each data point is a vector \(\mathbf{x}^{(i)}=[x^{(i)}_0, x^{(i)}_1,...,x^{(i)}_{n-1}]\).
Also, each data point has an associated label \(\mathbf{y} = [y^{(0)}, y^{(1)},..., y^{(m-1)}]\).
We will be doing binary classification so \(y^{(0)}\in \{0,1\}\)
d = pd.read_csv("local/data/moons.csv")
X = d[["col1", "col2"]].values
y = d["target"].values
print ("m=%d\nn=%d"%(X.shape[0], X.shape[1]))
X.shape, y.shape
m=200
n=2
((200, 2), (200,))
print (X[:10])
print (y[:10])
[[ 1.303374 -0.81478084]
[-0.98727894 -0.06456482]
[ 2.317555 0.44925394]
[ 0.85310268 -0.22083508]
[-0.97006406 0.64095693]
[ 1.65849049 -0.2342485 ]
[ 0.8684624 -0.28940167]
[ 0.05631143 0.00296565]
[ 0.88824669 -0.12888889]
[ 0.9925954 0.31451716]]
[1 0 1 1 0 1 1 1 1 0]
plt.scatter(X[:,0][y==0], X[:,1][y==0], color="red", label="class 0")
plt.scatter(X[:,0][y==1], X[:,1][y==1], color="blue", label="class 1")
plt.legend();
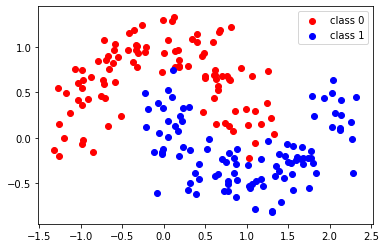
Task 1: Create a prediction function¶
complete the following prediction function which accepts:
\(\mathbf{\theta} = [\theta_0, \theta_1,... \theta_{n-1}] \in \mathbb{R}^n\), a
numpy
vector.\(b \in \mathbb{R}\) , a
float
.\(\mathbf{X} \in \mathbb{R}^{m\times n}\), a
numpy
2D array.
and returns
\(\hat{\mathbf{y}} = [\hat{y}^{(0)}, \hat{y}^{(1)}, ..., \hat{y}^{(m-1)}] \in \mathbb{R}^m\) defined as follows
with
note that we can also express the prediction vector \(\hat{\mathbf{y}}\) over the whole dataset as
observe that for any \(z\), the sigmoid function squeshes its value between 0 and 1. It can be simply seen as a continuous step function which converts any negative value into 0, and any positive value into 1.
challenge: use only one line of python code (not counting the \(\text{sigm}\) definition)
warn: your function must work with any \(\mathbf{X} \in \mathbb{R}^{m\times n}\) and \(\mathbf{\theta} \in \mathbb{R}^n\), for any \(m\) and \(n\), not only for \(n=2\)
z = np.linspace(-10,10,100)
sigm = lambda x: 1/(1+np.exp(-x))
plt.plot(z, sigm(z)); plt.grid(); plt.xlabel("z"); plt.ylabel("sigm(z)")
plt.axhline(0.5, color="black"); plt.axvline(0, color="black")
<matplotlib.lines.Line2D at 0x7f23f42aedd0>
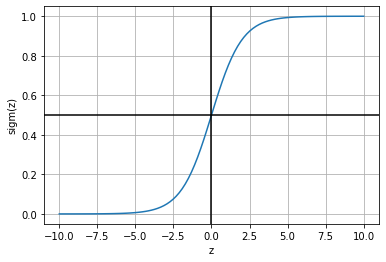
def prediction(t,b,X):
sigm = lambda z: 1/(1+np.exp(-z))
yhat = .... # YOUR CODE HERE
return yhat
check manually your code. The following should yield a vector with 200 elements, whose sum should be 99.97 at its four first elements
[0.02328672 0.91257015 0.03781132 0.16139629 ... ]
t,b = np.r_[[-2,2]], 0.5
yhat = prediction(t,b,X)
print (yhat.shape)
print (np.sum(yhat))
print (yhat)
submit your code
student.submit_task(globals(), task_id="task_01");
Task 2: Create the loss function¶
accepting the following paramters
\(\mathbf{\theta} = [\theta_0, \theta_1] \in \mathbb{R}^n\), a
numpy
vector.\(b \in \mathbb{R}\) , a
float
.\(\mathbf{X} \in \mathbb{R}^{m\times n}\), a
numpy
2D array.\(\mathbf{y} \in \mathbb{R}^m\), a
numpy
vector.
challenge: use only one line of python (not counting the sigm
and prediction
functions).
def loss(t,b,X,y):
sigm = lambda z: 1/(1+np.exp(-z))
result = ...
return result
check manually your code, you should get a loss of 0.664 approx.
t,b = np.r_[[-2,2]], 0.5
loss(t,b,X,y)
submit your code
student.submit_task(globals(), task_id="task_02");
Task 3: Obtain the best \(\theta\) and \(b\) with black box optimization¶
Complete the following function so that it returns the best \(\theta\) and \(b\) for a given data set.
Observe that
x0
inscipy.optimize.minimize
must be a vector of three items, the first two corresponding to \(\theta_0\) and \(\theta_1\), and the last one to \(b\).the return value of the optimizer
r.x
will also contain three items, and you will have to separate the first two intot
and the last one intob
.you will have to adapt your
loss
function created before so that it extracts \(\theta\) and \(b\) from the variableparams
.
def fit(X,y):
from scipy.optimize import minimize
def loss(params):
t = ... # extract t from params
b = ... # extract b params
sigm = lambda z: 1/(1+np.exp(-z))
return ... # your code here
tinit = np.zeros(shape=X.shape[1]+1) # keep this initialization
r = minimize(...) # complete the call to minimize
t = ... # extract t from r.x
b = ... # extract b from r.x
return t, b
t, b = fit(X,y)
check manually your code, you should get an accuracy of about 84%-87%, and a classification frontier similar to this one.
Observe how we convert the sigmoid output which can be any number \(\in [0,1]\), into a discrete classification (0 or 1) by thresholding on 0.5
from IPython.display import Image
Image(filename='local/imgs/logregmoons.png')
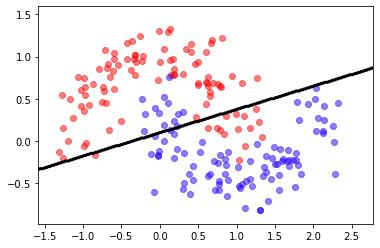
t, b
mlutils.plot_2Ddata_with_boundary(lambda X: prediction(t,b,X)>.5, X, y)
acc = np.mean( (prediction(t,b,X)>.5)==y)
print ("accuracy %.3f"%acc)
submit your code
student.submit_task(globals(), task_id="task_03");