05.04 - PARTICIPATE IN KAGGLE
Contents
05.04 - PARTICIPATE IN KAGGLE¶
!wget --no-cache -O init.py -q https://raw.githubusercontent.com/rramosp/ai4eng.v1/main/content/init.py
import init; init.init(force_download=False); init.get_weblink()
import numpy as np
import matplotlib.pyplot as plt
import tensorflow as tf
from sklearn.metrics import accuracy_score
from sklearn.model_selection import train_test_split
import local.lib.mlutils
import pandas as pd
from tensorflow.keras import Sequential
from tensorflow.keras.layers import Dense, Dropout, Flatten
%matplotlib inline
We use Titanic data in Kaggle¶
Register to Kaggle
Enter the competition Titanic Data at Kaggle
Download the
train.csv
andtest.csv
filesUPLOAD THE FILES to your notebook environment (in Colab, open the Files tab and upload)
d = pd.read_csv("train.csv")
print (d.shape)
(891, 12)
d.head()
PassengerId | Survived | Pclass | Name | Sex | Age | SibSp | Parch | Ticket | Fare | Cabin | Embarked | |
---|---|---|---|---|---|---|---|---|---|---|---|---|
0 | 1 | 0 | 3 | Braund, Mr. Owen Harris | male | 22.0 | 1 | 0 | A/5 21171 | 7.2500 | NaN | S |
1 | 2 | 1 | 1 | Cumings, Mrs. John Bradley (Florence Briggs Th... | female | 38.0 | 1 | 0 | PC 17599 | 71.2833 | C85 | C |
2 | 3 | 1 | 3 | Heikkinen, Miss. Laina | female | 26.0 | 0 | 0 | STON/O2. 3101282 | 7.9250 | NaN | S |
3 | 4 | 1 | 1 | Futrelle, Mrs. Jacques Heath (Lily May Peel) | female | 35.0 | 1 | 0 | 113803 | 53.1000 | C123 | S |
4 | 5 | 0 | 3 | Allen, Mr. William Henry | male | 35.0 | 0 | 0 | 373450 | 8.0500 | NaN | S |
Understand NaN
values are present
for i in d.columns:
print ("%20s"%i, np.sum(d[i].isna()))
PassengerId 0
Survived 0
Pclass 0
Name 0
Sex 0
Age 177
SibSp 0
Parch 0
Ticket 0
Fare 0
Cabin 687
Embarked 2
d.Embarked.value_counts()
S 644
C 168
Q 77
Name: Embarked, dtype: int64
plt.hist(d.Age.dropna().values, bins=30);
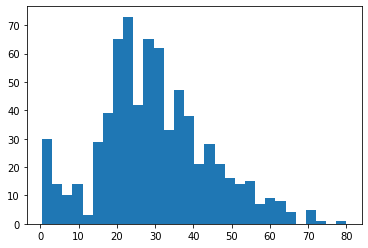
Remove uninformative columns
del(d["PassengerId"])
del(d["Name"])
del(d["Ticket"])
del(d["Cabin"])
Fix NaN
values
observe the different filling policies we decide to have
d["Embarked"] = d.Embarked.fillna("N")
d["Age"] = d.Age.fillna(d.Age.mean())
d.head()
Survived | Pclass | Sex | Age | SibSp | Parch | Fare | Embarked | |
---|---|---|---|---|---|---|---|---|
0 | 0 | 3 | male | 22.0 | 1 | 0 | 7.2500 | S |
1 | 1 | 1 | female | 38.0 | 1 | 0 | 71.2833 | C |
2 | 1 | 3 | female | 26.0 | 0 | 0 | 7.9250 | S |
3 | 1 | 1 | female | 35.0 | 1 | 0 | 53.1000 | S |
4 | 0 | 3 | male | 35.0 | 0 | 0 | 8.0500 | S |
plt.hist(d.Age.dropna().values, bins=30);
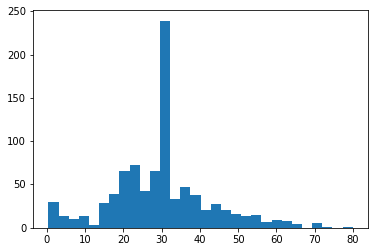
Turn categorical columns to a one_hot
encoding
def to_onehot(x):
values = np.unique(x)
r = np.r_[[np.argwhere(i==values)[0][0] for i in x]]
return np.eye(len(values))[r].astype(int)
k = to_onehot(d.Embarked.values)
k[:5]
array([[0, 0, 0, 1],
[1, 0, 0, 0],
[0, 0, 0, 1],
[0, 0, 0, 1],
[0, 0, 0, 1]])
def replace_columns_with_onehot(d, col):
k = to_onehot(d[col].values)
r = pd.DataFrame(k, columns=["%s_%d"%(col, i) for i in range(k.shape[1])], index=d.index).join(d)
del(r[col])
return r
d.head()
Survived | Pclass | Sex | Age | SibSp | Parch | Fare | Embarked | |
---|---|---|---|---|---|---|---|---|
0 | 0 | 3 | male | 22.0 | 1 | 0 | 7.2500 | S |
1 | 1 | 1 | female | 38.0 | 1 | 0 | 71.2833 | C |
2 | 1 | 3 | female | 26.0 | 0 | 0 | 7.9250 | S |
3 | 1 | 1 | female | 35.0 | 1 | 0 | 53.1000 | S |
4 | 0 | 3 | male | 35.0 | 0 | 0 | 8.0500 | S |
d = replace_columns_with_onehot(d, "Embarked")
d.head()
Embarked_0 | Embarked_1 | Embarked_2 | Embarked_3 | Survived | Pclass | Sex | Age | SibSp | Parch | Fare | |
---|---|---|---|---|---|---|---|---|---|---|---|
0 | 0 | 0 | 0 | 1 | 0 | 3 | male | 22.0 | 1 | 0 | 7.2500 |
1 | 1 | 0 | 0 | 0 | 1 | 1 | female | 38.0 | 1 | 0 | 71.2833 |
2 | 0 | 0 | 0 | 1 | 1 | 3 | female | 26.0 | 0 | 0 | 7.9250 |
3 | 0 | 0 | 0 | 1 | 1 | 1 | female | 35.0 | 1 | 0 | 53.1000 |
4 | 0 | 0 | 0 | 1 | 0 | 3 | male | 35.0 | 0 | 0 | 8.0500 |
d = replace_columns_with_onehot(d, "Sex")
d.head()
Sex_0 | Sex_1 | Embarked_0 | Embarked_1 | Embarked_2 | Embarked_3 | Survived | Pclass | Age | SibSp | Parch | Fare | |
---|---|---|---|---|---|---|---|---|---|---|---|---|
0 | 0 | 1 | 0 | 0 | 0 | 1 | 0 | 3 | 22.0 | 1 | 0 | 7.2500 |
1 | 1 | 0 | 1 | 0 | 0 | 0 | 1 | 1 | 38.0 | 1 | 0 | 71.2833 |
2 | 1 | 0 | 0 | 0 | 0 | 1 | 1 | 3 | 26.0 | 0 | 0 | 7.9250 |
3 | 1 | 0 | 0 | 0 | 0 | 1 | 1 | 1 | 35.0 | 1 | 0 | 53.1000 |
4 | 0 | 1 | 0 | 0 | 0 | 1 | 0 | 3 | 35.0 | 0 | 0 | 8.0500 |
d.shape, d.values.sum()
((891, 12), 60142.86312352941)
Put all transformations together¶
def clean_titanic(d):
del(d["PassengerId"])
del(d["Name"])
del(d["Ticket"])
del(d["Cabin"])
d["Embarked"] = d.Embarked.fillna("N")
d["Fare"] = d.Fare.fillna(d.Fare.mean())
d["Age"] = d.Age.fillna(d.Age.mean())
d = replace_columns_with_onehot(d, "Embarked")
d = replace_columns_with_onehot(d, "Sex")
return d
transform train and test data together
observe that test data does not have a
Survival
column. This is the result to submit to Kaggle
dtr = pd.read_csv("train.csv")
dts = pd.read_csv("test.csv")
lentr = len(dtr)
dtr.shape, dts.shape
((891, 12), (418, 11))
dts.head()
PassengerId | Pclass | Name | Sex | Age | SibSp | Parch | Ticket | Fare | Cabin | Embarked | |
---|---|---|---|---|---|---|---|---|---|---|---|
0 | 892 | 3 | Kelly, Mr. James | male | 34.5 | 0 | 0 | 330911 | 7.8292 | NaN | Q |
1 | 893 | 3 | Wilkes, Mrs. James (Ellen Needs) | female | 47.0 | 1 | 0 | 363272 | 7.0000 | NaN | S |
2 | 894 | 2 | Myles, Mr. Thomas Francis | male | 62.0 | 0 | 0 | 240276 | 9.6875 | NaN | Q |
3 | 895 | 3 | Wirz, Mr. Albert | male | 27.0 | 0 | 0 | 315154 | 8.6625 | NaN | S |
4 | 896 | 3 | Hirvonen, Mrs. Alexander (Helga E Lindqvist) | female | 22.0 | 1 | 1 | 3101298 | 12.2875 | NaN | S |
get data ready for training
source_cols = [i for i in dtr.columns if i!="Survived"]
all_data = pd.concat((dtr[source_cols], dts[source_cols]))
all_data.index = range(len(all_data))
all_data = clean_titanic(all_data)
Xtr, ytr = all_data.iloc[:lentr].values, dtr["Survived"].values
Xts = all_data.iloc[lentr:].values
print (Xtr.shape, ytr.shape)
print (Xts.shape)
(891, 11) (891,)
(418, 11)
cross validate for model selection
from sklearn.ensemble import RandomForestClassifier
from sklearn.svm import SVC
from sklearn.model_selection import cross_val_score
rf = RandomForestClassifier()
print (cross_val_score(rf, Xtr, ytr))
svc = SVC()
print (cross_val_score(svc, Xtr, ytr))
[0.77094972 0.81460674 0.84831461 0.79213483 0.83707865]
[0.59217877 0.71348315 0.69101124 0.68539326 0.69101124]
now train with full dataset and generate submission for Kaggle
rf.fit(Xtr, ytr)
preds_ts = rf.predict(Xts)
preds_ts
array([0, 0, 0, 1, 0, 0, 0, 0, 1, 0, 0, 0, 1, 0, 1, 1, 0, 1, 0, 1, 1, 1,
1, 1, 1, 0, 1, 1, 1, 0, 0, 0, 1, 0, 1, 1, 0, 0, 0, 1, 0, 1, 0, 1,
1, 0, 0, 0, 1, 1, 0, 0, 1, 1, 0, 0, 0, 0, 0, 1, 0, 0, 0, 1, 1, 1,
1, 0, 0, 1, 1, 0, 0, 0, 1, 0, 0, 1, 0, 1, 1, 0, 0, 0, 0, 0, 1, 0,
1, 1, 0, 0, 1, 0, 1, 0, 1, 0, 0, 0, 1, 0, 0, 0, 1, 0, 0, 0, 0, 0,
0, 1, 1, 0, 1, 0, 0, 1, 0, 1, 1, 0, 1, 0, 0, 1, 0, 1, 0, 0, 1, 0,
0, 0, 0, 0, 0, 0, 0, 0, 0, 1, 0, 0, 1, 0, 0, 0, 1, 0, 1, 0, 0, 1,
0, 0, 1, 1, 1, 1, 1, 1, 1, 0, 0, 0, 0, 0, 1, 0, 0, 0, 0, 0, 0, 1,
1, 1, 1, 1, 0, 1, 1, 0, 1, 0, 1, 0, 0, 0, 0, 0, 1, 0, 1, 0, 1, 0,
0, 0, 1, 1, 0, 1, 0, 0, 0, 0, 1, 0, 0, 0, 0, 0, 1, 0, 1, 0, 1, 0,
1, 0, 1, 1, 1, 0, 0, 1, 0, 0, 0, 1, 0, 0, 1, 0, 1, 1, 1, 1, 1, 1,
0, 0, 0, 0, 1, 0, 1, 0, 1, 0, 0, 0, 0, 0, 0, 0, 1, 0, 0, 0, 1, 1,
0, 0, 0, 0, 0, 0, 0, 0, 1, 1, 0, 1, 0, 0, 0, 0, 0, 1, 1, 0, 1, 0,
0, 0, 0, 0, 0, 0, 0, 0, 0, 0, 1, 0, 0, 0, 0, 0, 0, 0, 1, 1, 1, 1,
0, 0, 0, 1, 0, 0, 1, 1, 0, 0, 0, 0, 0, 0, 0, 1, 1, 0, 1, 0, 0, 0,
1, 1, 0, 0, 0, 0, 1, 0, 0, 0, 0, 0, 0, 1, 0, 1, 0, 1, 0, 1, 1, 0,
0, 0, 1, 0, 1, 0, 0, 1, 0, 1, 1, 1, 1, 0, 0, 0, 1, 1, 0, 1, 0, 0,
1, 1, 0, 0, 0, 0, 0, 0, 1, 0, 1, 1, 0, 0, 0, 0, 0, 1, 0, 0, 0, 1,
0, 1, 0, 0, 1, 0, 1, 0, 0, 0, 0, 0, 1, 1, 1, 1, 0, 0, 1, 0, 0, 1])
get predictions ready to submit to Kaggle
see https://www.kaggle.com/c/titanic#evaluation for file format
submission = pd.DataFrame([dts.PassengerId, pd.Series(preds_ts, name="Survived")]).T
submission.head()
PassengerId | Survived | |
---|---|---|
0 | 892 | 0 |
1 | 893 | 0 |
2 | 894 | 0 |
3 | 895 | 1 |
4 | 896 | 0 |
submission.to_csv("titanic_kaggle.csv", index=False)
!head titanic_kaggle.csv
PassengerId,Survived
892,0
893,0
894,0
895,1
896,0
897,0
898,0
899,0
900,1