04.01 - DATA EXPLORATION
Contents
04.01 - DATA EXPLORATION¶
!wget --no-cache -O init.py -q https://raw.githubusercontent.com/rramosp/ai4eng.v1/main/content/init.py
import init; init.init(force_download=False); init.get_weblink()
Based on Kaggle House Pricing Prediction Competition¶
Inspect and learn from the competition Notebooks
You must make available to this notebook the
train.csv
file from the competition data section. If running this notebook in Google Colab you must upload it in the notebook files section in Colab.
## KEEPOUTPUT
import pandas as pd
import seaborn as sns
import numpy as np
import matplotlib.pyplot as plt
%matplotlib inline
d = pd.read_csv("train.csv")
d.head()
Id | MSSubClass | MSZoning | LotFrontage | LotArea | Street | Alley | LotShape | LandContour | Utilities | ... | PoolArea | PoolQC | Fence | MiscFeature | MiscVal | MoSold | YrSold | SaleType | SaleCondition | SalePrice | |
---|---|---|---|---|---|---|---|---|---|---|---|---|---|---|---|---|---|---|---|---|---|
0 | 1 | 60 | RL | 65.0 | 8450 | Pave | NaN | Reg | Lvl | AllPub | ... | 0 | NaN | NaN | NaN | 0 | 2 | 2008 | WD | Normal | 208500 |
1 | 2 | 20 | RL | 80.0 | 9600 | Pave | NaN | Reg | Lvl | AllPub | ... | 0 | NaN | NaN | NaN | 0 | 5 | 2007 | WD | Normal | 181500 |
2 | 3 | 60 | RL | 68.0 | 11250 | Pave | NaN | IR1 | Lvl | AllPub | ... | 0 | NaN | NaN | NaN | 0 | 9 | 2008 | WD | Normal | 223500 |
3 | 4 | 70 | RL | 60.0 | 9550 | Pave | NaN | IR1 | Lvl | AllPub | ... | 0 | NaN | NaN | NaN | 0 | 2 | 2006 | WD | Abnorml | 140000 |
4 | 5 | 60 | RL | 84.0 | 14260 | Pave | NaN | IR1 | Lvl | AllPub | ... | 0 | NaN | NaN | NaN | 0 | 12 | 2008 | WD | Normal | 250000 |
5 rows × 81 columns
data size
## KEEPOUTPUT
print (d.shape)
(1460, 81)
Missing values in columns
## KEEPOUTPUT
k = d.isna().sum()
k[k!=0]
LotFrontage 259
Alley 1369
MasVnrType 8
MasVnrArea 8
BsmtQual 37
BsmtCond 37
BsmtExposure 38
BsmtFinType1 37
BsmtFinType2 38
Electrical 1
FireplaceQu 690
GarageType 81
GarageYrBlt 81
GarageFinish 81
GarageQual 81
GarageCond 81
PoolQC 1453
Fence 1179
MiscFeature 1406
dtype: int64
Inspect the target variable¶
## KEEPOUTPUT
sns.distplot(d['SalePrice']);
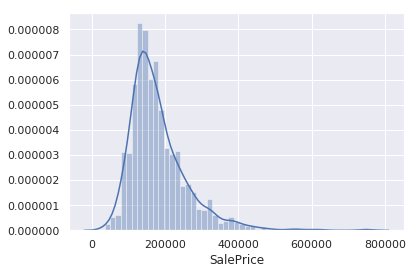
Discover data types¶
## KEEPOUTPUT
d.columns
Index(['Id', 'MSSubClass', 'MSZoning', 'LotFrontage', 'LotArea', 'Street',
'Alley', 'LotShape', 'LandContour', 'Utilities', 'LotConfig',
'LandSlope', 'Neighborhood', 'Condition1', 'Condition2', 'BldgType',
'HouseStyle', 'OverallQual', 'OverallCond', 'YearBuilt', 'YearRemodAdd',
'RoofStyle', 'RoofMatl', 'Exterior1st', 'Exterior2nd', 'MasVnrType',
'MasVnrArea', 'ExterQual', 'ExterCond', 'Foundation', 'BsmtQual',
'BsmtCond', 'BsmtExposure', 'BsmtFinType1', 'BsmtFinSF1',
'BsmtFinType2', 'BsmtFinSF2', 'BsmtUnfSF', 'TotalBsmtSF', 'Heating',
'HeatingQC', 'CentralAir', 'Electrical', '1stFlrSF', '2ndFlrSF',
'LowQualFinSF', 'GrLivArea', 'BsmtFullBath', 'BsmtHalfBath', 'FullBath',
'HalfBath', 'BedroomAbvGr', 'KitchenAbvGr', 'KitchenQual',
'TotRmsAbvGrd', 'Functional', 'Fireplaces', 'FireplaceQu', 'GarageType',
'GarageYrBlt', 'GarageFinish', 'GarageCars', 'GarageArea', 'GarageQual',
'GarageCond', 'PavedDrive', 'WoodDeckSF', 'OpenPorchSF',
'EnclosedPorch', '3SsnPorch', 'ScreenPorch', 'PoolArea', 'PoolQC',
'Fence', 'MiscFeature', 'MiscVal', 'MoSold', 'YrSold', 'SaleType',
'SaleCondition', 'SalePrice'],
dtype='object')
## KEEPOUTPUT
for c in d.columns:
print ("%20s"%c, d[c].dtype)
Id int64
MSSubClass int64
MSZoning object
LotFrontage float64
LotArea int64
Street object
Alley object
LotShape object
LandContour object
Utilities object
LotConfig object
LandSlope object
Neighborhood object
Condition1 object
Condition2 object
BldgType object
HouseStyle object
OverallQual int64
OverallCond int64
YearBuilt int64
YearRemodAdd int64
RoofStyle object
RoofMatl object
Exterior1st object
Exterior2nd object
MasVnrType object
MasVnrArea float64
ExterQual object
ExterCond object
Foundation object
BsmtQual object
BsmtCond object
BsmtExposure object
BsmtFinType1 object
BsmtFinSF1 int64
BsmtFinType2 object
BsmtFinSF2 int64
BsmtUnfSF int64
TotalBsmtSF int64
Heating object
HeatingQC object
CentralAir object
Electrical object
1stFlrSF int64
2ndFlrSF int64
LowQualFinSF int64
GrLivArea int64
BsmtFullBath int64
BsmtHalfBath int64
FullBath int64
HalfBath int64
BedroomAbvGr int64
KitchenAbvGr int64
KitchenQual object
TotRmsAbvGrd int64
Functional object
Fireplaces int64
FireplaceQu object
GarageType object
GarageYrBlt float64
GarageFinish object
GarageCars int64
GarageArea int64
GarageQual object
GarageCond object
PavedDrive object
WoodDeckSF int64
OpenPorchSF int64
EnclosedPorch int64
3SsnPorch int64
ScreenPorch int64
PoolArea int64
PoolQC object
Fence object
MiscFeature object
MiscVal int64
MoSold int64
YrSold int64
SaleType object
SaleCondition object
SalePrice int64
Inspect numeric columns¶
## KEEPOUTPUT
d._get_numeric_data().describe().T
count | mean | std | min | 25% | 50% | 75% | max | |
---|---|---|---|---|---|---|---|---|
Id | 1460.0 | 730.500000 | 421.610009 | 1.0 | 365.75 | 730.5 | 1095.25 | 1460.0 |
MSSubClass | 1460.0 | 56.897260 | 42.300571 | 20.0 | 20.00 | 50.0 | 70.00 | 190.0 |
LotFrontage | 1201.0 | 70.049958 | 24.284752 | 21.0 | 59.00 | 69.0 | 80.00 | 313.0 |
LotArea | 1460.0 | 10516.828082 | 9981.264932 | 1300.0 | 7553.50 | 9478.5 | 11601.50 | 215245.0 |
OverallQual | 1460.0 | 6.099315 | 1.382997 | 1.0 | 5.00 | 6.0 | 7.00 | 10.0 |
OverallCond | 1460.0 | 5.575342 | 1.112799 | 1.0 | 5.00 | 5.0 | 6.00 | 9.0 |
YearBuilt | 1460.0 | 1971.267808 | 30.202904 | 1872.0 | 1954.00 | 1973.0 | 2000.00 | 2010.0 |
YearRemodAdd | 1460.0 | 1984.865753 | 20.645407 | 1950.0 | 1967.00 | 1994.0 | 2004.00 | 2010.0 |
MasVnrArea | 1452.0 | 103.685262 | 181.066207 | 0.0 | 0.00 | 0.0 | 166.00 | 1600.0 |
BsmtFinSF1 | 1460.0 | 443.639726 | 456.098091 | 0.0 | 0.00 | 383.5 | 712.25 | 5644.0 |
BsmtFinSF2 | 1460.0 | 46.549315 | 161.319273 | 0.0 | 0.00 | 0.0 | 0.00 | 1474.0 |
BsmtUnfSF | 1460.0 | 567.240411 | 441.866955 | 0.0 | 223.00 | 477.5 | 808.00 | 2336.0 |
TotalBsmtSF | 1460.0 | 1057.429452 | 438.705324 | 0.0 | 795.75 | 991.5 | 1298.25 | 6110.0 |
1stFlrSF | 1460.0 | 1162.626712 | 386.587738 | 334.0 | 882.00 | 1087.0 | 1391.25 | 4692.0 |
2ndFlrSF | 1460.0 | 346.992466 | 436.528436 | 0.0 | 0.00 | 0.0 | 728.00 | 2065.0 |
LowQualFinSF | 1460.0 | 5.844521 | 48.623081 | 0.0 | 0.00 | 0.0 | 0.00 | 572.0 |
GrLivArea | 1460.0 | 1515.463699 | 525.480383 | 334.0 | 1129.50 | 1464.0 | 1776.75 | 5642.0 |
BsmtFullBath | 1460.0 | 0.425342 | 0.518911 | 0.0 | 0.00 | 0.0 | 1.00 | 3.0 |
BsmtHalfBath | 1460.0 | 0.057534 | 0.238753 | 0.0 | 0.00 | 0.0 | 0.00 | 2.0 |
FullBath | 1460.0 | 1.565068 | 0.550916 | 0.0 | 1.00 | 2.0 | 2.00 | 3.0 |
HalfBath | 1460.0 | 0.382877 | 0.502885 | 0.0 | 0.00 | 0.0 | 1.00 | 2.0 |
BedroomAbvGr | 1460.0 | 2.866438 | 0.815778 | 0.0 | 2.00 | 3.0 | 3.00 | 8.0 |
KitchenAbvGr | 1460.0 | 1.046575 | 0.220338 | 0.0 | 1.00 | 1.0 | 1.00 | 3.0 |
TotRmsAbvGrd | 1460.0 | 6.517808 | 1.625393 | 2.0 | 5.00 | 6.0 | 7.00 | 14.0 |
Fireplaces | 1460.0 | 0.613014 | 0.644666 | 0.0 | 0.00 | 1.0 | 1.00 | 3.0 |
GarageYrBlt | 1379.0 | 1978.506164 | 24.689725 | 1900.0 | 1961.00 | 1980.0 | 2002.00 | 2010.0 |
GarageCars | 1460.0 | 1.767123 | 0.747315 | 0.0 | 1.00 | 2.0 | 2.00 | 4.0 |
GarageArea | 1460.0 | 472.980137 | 213.804841 | 0.0 | 334.50 | 480.0 | 576.00 | 1418.0 |
WoodDeckSF | 1460.0 | 94.244521 | 125.338794 | 0.0 | 0.00 | 0.0 | 168.00 | 857.0 |
OpenPorchSF | 1460.0 | 46.660274 | 66.256028 | 0.0 | 0.00 | 25.0 | 68.00 | 547.0 |
EnclosedPorch | 1460.0 | 21.954110 | 61.119149 | 0.0 | 0.00 | 0.0 | 0.00 | 552.0 |
3SsnPorch | 1460.0 | 3.409589 | 29.317331 | 0.0 | 0.00 | 0.0 | 0.00 | 508.0 |
ScreenPorch | 1460.0 | 15.060959 | 55.757415 | 0.0 | 0.00 | 0.0 | 0.00 | 480.0 |
PoolArea | 1460.0 | 2.758904 | 40.177307 | 0.0 | 0.00 | 0.0 | 0.00 | 738.0 |
MiscVal | 1460.0 | 43.489041 | 496.123024 | 0.0 | 0.00 | 0.0 | 0.00 | 15500.0 |
MoSold | 1460.0 | 6.321918 | 2.703626 | 1.0 | 5.00 | 6.0 | 8.00 | 12.0 |
YrSold | 1460.0 | 2007.815753 | 1.328095 | 2006.0 | 2007.00 | 2008.0 | 2009.00 | 2010.0 |
SalePrice | 1460.0 | 180921.195890 | 79442.502883 | 34900.0 | 129975.00 | 163000.0 | 214000.00 | 755000.0 |
## KEEPOUTPUT
cols = ['OverallQual', 'GrLivArea', 'GarageCars', 'TotalBsmtSF', 'FullBath', 'YearBuilt', 'SalePrice']
#cols = np.unique(list(np.random.permutation(d._get_numeric_data().columns)[:5])+['SalePrice'])
sns.set()
sns.pairplot(d[cols])
<seaborn.axisgrid.PairGrid at 0x7f9ef2534e10>
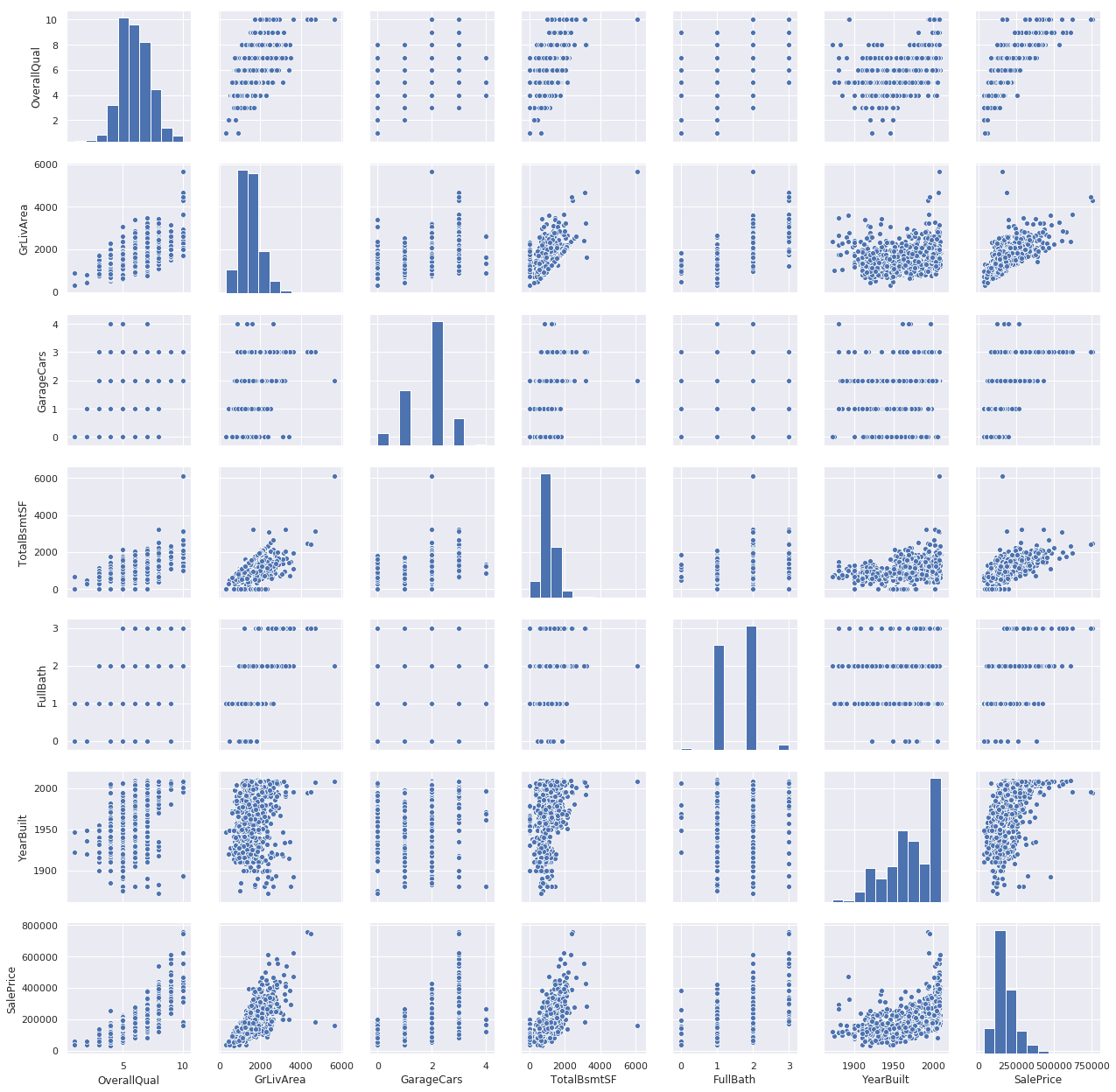
correlations¶
## KEEPOUTPUT
#correlation matrix
corrmat = d.corr()
f, ax = plt.subplots(figsize=(12, 9))
sns.heatmap(corrmat, vmax=.8, square=True);
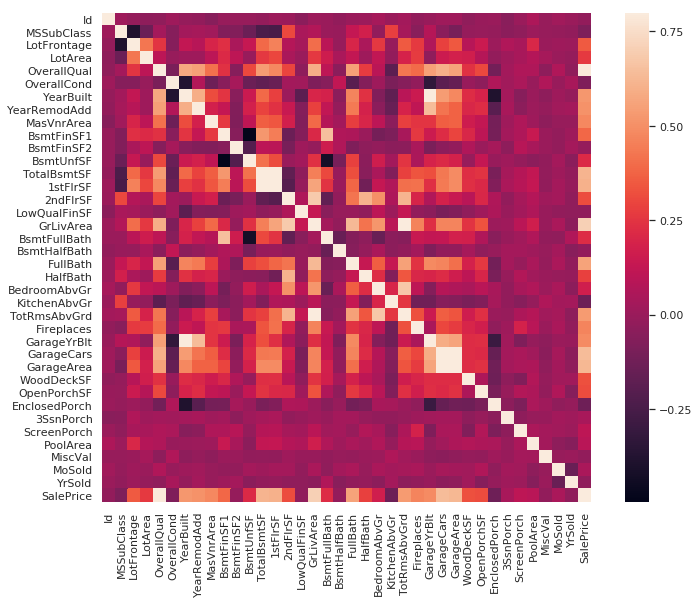
Inspect categorical variables¶
## KEEPOUTPUT
ccols = [i for i in d.columns if not i in d._get_numeric_data()]
print (ccols)
['MSZoning', 'Street', 'Alley', 'LotShape', 'LandContour', 'Utilities', 'LotConfig', 'LandSlope', 'Neighborhood', 'Condition1', 'Condition2', 'BldgType', 'HouseStyle', 'RoofStyle', 'RoofMatl', 'Exterior1st', 'Exterior2nd', 'MasVnrType', 'ExterQual', 'ExterCond', 'Foundation', 'BsmtQual', 'BsmtCond', 'BsmtExposure', 'BsmtFinType1', 'BsmtFinType2', 'Heating', 'HeatingQC', 'CentralAir', 'Electrical', 'KitchenQual', 'Functional', 'FireplaceQu', 'GarageType', 'GarageFinish', 'GarageQual', 'GarageCond', 'PavedDrive', 'PoolQC', 'Fence', 'MiscFeature', 'SaleType', 'SaleCondition']
## KEEPOUTPUT
for c in ccols:
print ("%10s"%c, np.unique(d[c].dropna()))
MSZoning ['C (all)' 'FV' 'RH' 'RL' 'RM']
Street ['Grvl' 'Pave']
Alley ['Grvl' 'Pave']
LotShape ['IR1' 'IR2' 'IR3' 'Reg']
LandContour ['Bnk' 'HLS' 'Low' 'Lvl']
Utilities ['AllPub' 'NoSeWa']
LotConfig ['Corner' 'CulDSac' 'FR2' 'FR3' 'Inside']
LandSlope ['Gtl' 'Mod' 'Sev']
Neighborhood ['Blmngtn' 'Blueste' 'BrDale' 'BrkSide' 'ClearCr' 'CollgCr' 'Crawfor'
'Edwards' 'Gilbert' 'IDOTRR' 'MeadowV' 'Mitchel' 'NAmes' 'NPkVill'
'NWAmes' 'NoRidge' 'NridgHt' 'OldTown' 'SWISU' 'Sawyer' 'SawyerW'
'Somerst' 'StoneBr' 'Timber' 'Veenker']
Condition1 ['Artery' 'Feedr' 'Norm' 'PosA' 'PosN' 'RRAe' 'RRAn' 'RRNe' 'RRNn']
Condition2 ['Artery' 'Feedr' 'Norm' 'PosA' 'PosN' 'RRAe' 'RRAn' 'RRNn']
BldgType ['1Fam' '2fmCon' 'Duplex' 'Twnhs' 'TwnhsE']
HouseStyle ['1.5Fin' '1.5Unf' '1Story' '2.5Fin' '2.5Unf' '2Story' 'SFoyer' 'SLvl']
RoofStyle ['Flat' 'Gable' 'Gambrel' 'Hip' 'Mansard' 'Shed']
RoofMatl ['ClyTile' 'CompShg' 'Membran' 'Metal' 'Roll' 'Tar&Grv' 'WdShake'
'WdShngl']
Exterior1st ['AsbShng' 'AsphShn' 'BrkComm' 'BrkFace' 'CBlock' 'CemntBd' 'HdBoard'
'ImStucc' 'MetalSd' 'Plywood' 'Stone' 'Stucco' 'VinylSd' 'Wd Sdng'
'WdShing']
Exterior2nd ['AsbShng' 'AsphShn' 'Brk Cmn' 'BrkFace' 'CBlock' 'CmentBd' 'HdBoard'
'ImStucc' 'MetalSd' 'Other' 'Plywood' 'Stone' 'Stucco' 'VinylSd'
'Wd Sdng' 'Wd Shng']
MasVnrType ['BrkCmn' 'BrkFace' 'None' 'Stone']
ExterQual ['Ex' 'Fa' 'Gd' 'TA']
ExterCond ['Ex' 'Fa' 'Gd' 'Po' 'TA']
Foundation ['BrkTil' 'CBlock' 'PConc' 'Slab' 'Stone' 'Wood']
BsmtQual ['Ex' 'Fa' 'Gd' 'TA']
BsmtCond ['Fa' 'Gd' 'Po' 'TA']
BsmtExposure ['Av' 'Gd' 'Mn' 'No']
BsmtFinType1 ['ALQ' 'BLQ' 'GLQ' 'LwQ' 'Rec' 'Unf']
BsmtFinType2 ['ALQ' 'BLQ' 'GLQ' 'LwQ' 'Rec' 'Unf']
Heating ['Floor' 'GasA' 'GasW' 'Grav' 'OthW' 'Wall']
HeatingQC ['Ex' 'Fa' 'Gd' 'Po' 'TA']
CentralAir ['N' 'Y']
Electrical ['FuseA' 'FuseF' 'FuseP' 'Mix' 'SBrkr']
KitchenQual ['Ex' 'Fa' 'Gd' 'TA']
Functional ['Maj1' 'Maj2' 'Min1' 'Min2' 'Mod' 'Sev' 'Typ']
FireplaceQu ['Ex' 'Fa' 'Gd' 'Po' 'TA']
GarageType ['2Types' 'Attchd' 'Basment' 'BuiltIn' 'CarPort' 'Detchd']
GarageFinish ['Fin' 'RFn' 'Unf']
GarageQual ['Ex' 'Fa' 'Gd' 'Po' 'TA']
GarageCond ['Ex' 'Fa' 'Gd' 'Po' 'TA']
PavedDrive ['N' 'P' 'Y']
PoolQC ['Ex' 'Fa' 'Gd']
Fence ['GdPrv' 'GdWo' 'MnPrv' 'MnWw']
MiscFeature ['Gar2' 'Othr' 'Shed' 'TenC']
SaleType ['COD' 'CWD' 'Con' 'ConLD' 'ConLI' 'ConLw' 'New' 'Oth' 'WD']
SaleCondition ['Abnorml' 'AdjLand' 'Alloca' 'Family' 'Normal' 'Partial']
## KEEPOUTPUT
c="GarageType"
d[c].value_counts()
Attchd 870
Detchd 387
BuiltIn 88
Basment 19
CarPort 9
2Types 6
Name: GarageType, dtype: int64
## KEEPOUTPUT
plt.figure(figsize=(20,8))
for i,c in enumerate(["ExterQual", "HouseStyle", "LandSlope", "Alley"]):
plt.subplot(2,4,i+1)
k=d[[c,"SalePrice"]].dropna()
for v in d[c].dropna().unique():
sns.distplot(k.SalePrice[k[c]==v], label=v);
plt.title(c)
plt.yticks([])
plt.legend()
plt.subplot(2,4,i+5)
vc = k[c].value_counts()
sns.barplot(vc.index, vc.values)
plt.xticks(range(len(vc)), vc.index, rotation="vertical")
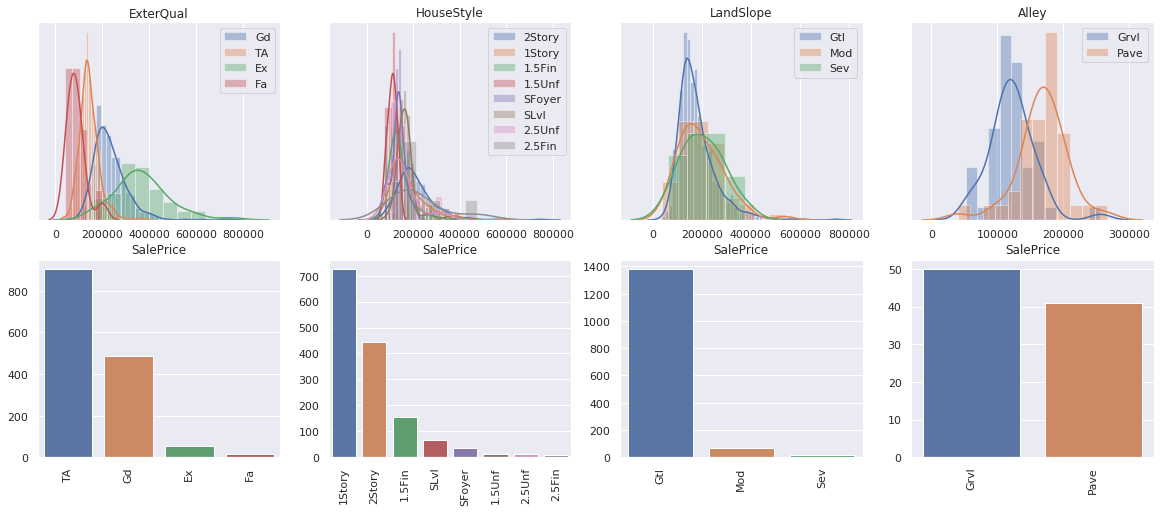
Vision on mission values¶
Missing values in columns
## KEEPOUTPUT
k = d.isna().sum()
k[k!=0]
LotFrontage 259
Alley 1369
MasVnrType 8
MasVnrArea 8
BsmtQual 37
BsmtCond 37
BsmtExposure 38
BsmtFinType1 37
BsmtFinType2 38
Electrical 1
FireplaceQu 690
GarageType 81
GarageYrBlt 81
GarageFinish 81
GarageQual 81
GarageCond 81
PoolQC 1453
Fence 1179
MiscFeature 1406
dtype: int64
## KEEPOUTPUT
ax = plt.figure(figsize=(30,15)).add_subplot(111)
ax.imshow(d.isna().values.T)
ax.set_aspect(12)
plt.yticks(range(d.shape[1]), d.columns);
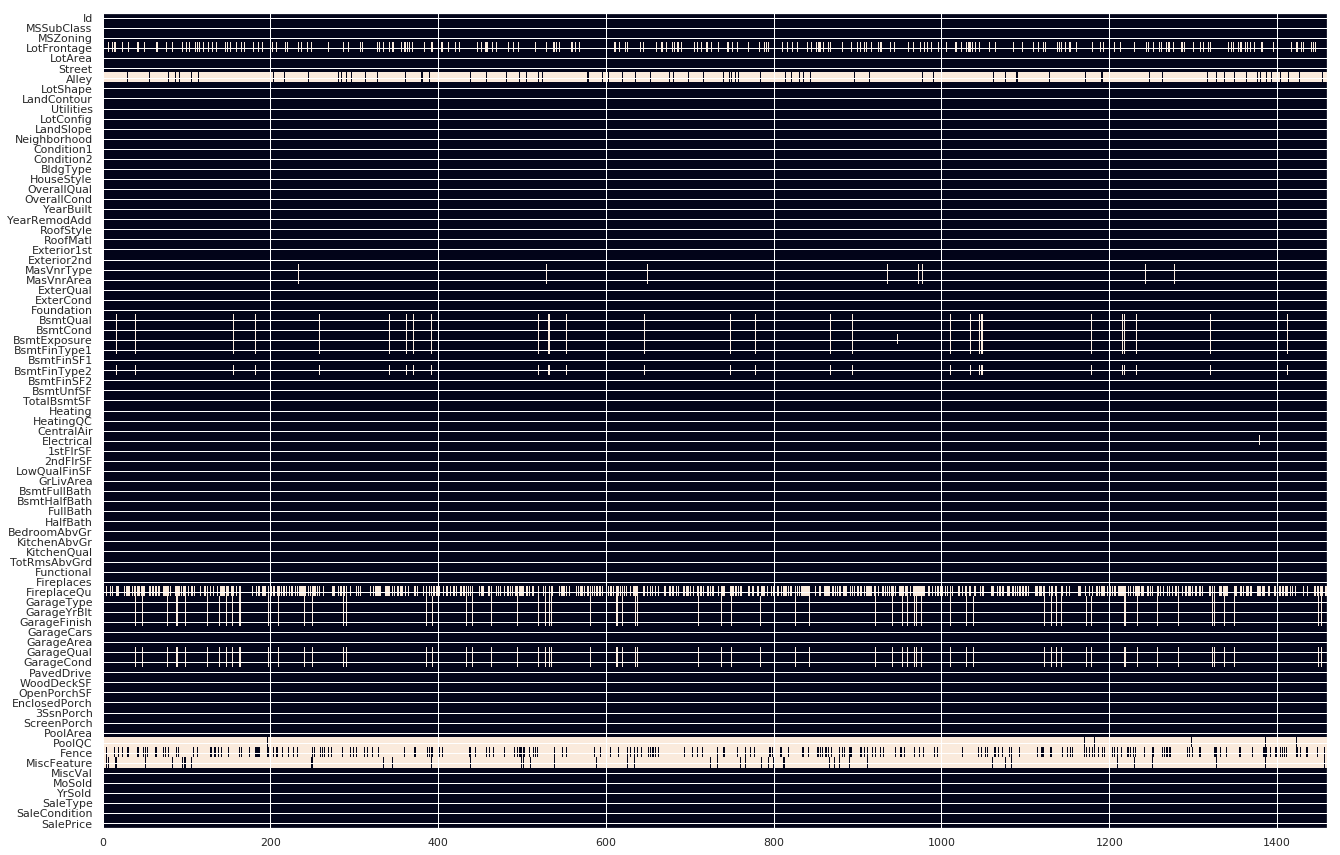