02.04 - PANDAS
Contents
02.04 - PANDAS¶
!wget --no-cache -O init.py -q https://raw.githubusercontent.com/rramosp/ai4eng.v1/main/content/init.py
import init; init.init(force_download=False); init.get_weblink()
import numpy as np
import pandas as pd
import matplotlib.pyplot as plt
%matplotlib inline
pandas
is mostly about manipulating tables of data¶
see this cheat sheet: https://pandas.pydata.org/Pandas_Cheat_Sheet.pdf
Pandas main object is a DataFrame
¶
can read .csv, .excel, etc.
!head local/data/internet_facebook.dat
# Pais,Uso_Internet,Uso_Facebook
Argentina,49.40,30.53
Australia,80.60,46.01
Belgium,67.30,36.98
Brazil,37.76,4.39
Canada,72.30,52.08
Chile,50.90,46.14
China,22.40,0.05
Colombia,38.80,25.90
Egypt,12.90,5.68
!wc local/data/weather_data_austin_2010.csv
8760 17519 254046 local/data/weather_data_austin_2010.csv
df = pd.read_csv('local/data/internet_facebook.dat', index_col='# Pais')
df
Uso_Internet | Uso_Facebook | |
---|---|---|
# Pais | ||
Argentina | 49.40 | 30.53 |
Australia | 80.60 | 46.01 |
Belgium | 67.30 | 36.98 |
Brazil | 37.76 | 4.39 |
Canada | 72.30 | 52.08 |
Chile | 50.90 | 46.14 |
China | 22.40 | 0.05 |
Colombia | 38.80 | 25.90 |
Egypt | 12.90 | 5.68 |
France | 65.70 | 32.91 |
Germany | 67.00 | 14.07 |
Hong_Kong | 69.50 | 52.33 |
India | 7.10 | 1.52 |
Indonesia | 10.50 | 13.49 |
Italy | 48.80 | 30.62 |
Japan | 73.80 | 2.00 |
Malaysia | 62.80 | 37.77 |
Mexico | 24.90 | 16.80 |
Netherlands | 82.90 | 20.54 |
Peru | 26.20 | 13.34 |
Philippines | 21.50 | 19.68 |
Poland | 52.00 | 11.79 |
Russia | 27.00 | 2.99 |
Saudi_Arabia | 22.70 | 11.65 |
South_Africa | 10.50 | 7.83 |
Spain | 66.80 | 30.24 |
Sweden | 80.70 | 44.72 |
Taiwan | 66.10 | 38.21 |
Thailand | 20.50 | 10.29 |
Turkey | 35.00 | 31.91 |
USA | 77.33 | 46.98 |
UK | 70.18 | 45.97 |
Venezuela | 25.50 | 28.64 |
df.head()
Uso_Internet | Uso_Facebook | |
---|---|---|
# Pais | ||
Argentina | 49.40 | 30.53 |
Australia | 80.60 | 46.01 |
Belgium | 67.30 | 36.98 |
Brazil | 37.76 | 4.39 |
Canada | 72.30 | 52.08 |
df.tail()
Uso_Internet | Uso_Facebook | |
---|---|---|
# Pais | ||
Thailand | 20.50 | 10.29 |
Turkey | 35.00 | 31.91 |
USA | 77.33 | 46.98 |
UK | 70.18 | 45.97 |
Venezuela | 25.50 | 28.64 |
df.columns
Index(['Uso_Internet', 'Uso_Facebook'], dtype='object')
df.index
Index(['Argentina', 'Australia', 'Belgium', 'Brazil', 'Canada', 'Chile',
'China', 'Colombia', 'Egypt', 'France', 'Germany', 'Hong_Kong', 'India',
'Indonesia', 'Italy', 'Japan', 'Malaysia', 'Mexico', 'Netherlands',
'Peru', 'Philippines', 'Poland', 'Russia', 'Saudi_Arabia',
'South_Africa', 'Spain', 'Sweden', 'Taiwan', 'Thailand', 'Turkey',
'USA', 'UK', 'Venezuela'],
dtype='object', name='# Pais')
fix the index name
df.index.name="Pais"
df.head()
Uso_Internet | Uso_Facebook | |
---|---|---|
Pais | ||
Argentina | 49.40 | 30.53 |
Australia | 80.60 | 46.01 |
Belgium | 67.30 | 36.98 |
Brazil | 37.76 | 4.39 |
Canada | 72.30 | 52.08 |
df.describe()
Uso_Internet | Uso_Facebook | |
---|---|---|
count | 33.000000 | 33.000000 |
mean | 46.890000 | 24.668182 |
std | 24.456421 | 16.511662 |
min | 7.100000 | 0.050000 |
25% | 24.900000 | 11.650000 |
50% | 49.400000 | 25.900000 |
75% | 67.300000 | 37.770000 |
max | 82.900000 | 52.330000 |
df.info()
<class 'pandas.core.frame.DataFrame'>
Index: 33 entries, Argentina to Venezuela
Data columns (total 2 columns):
Uso_Internet 33 non-null float64
Uso_Facebook 33 non-null float64
dtypes: float64(2)
memory usage: 792.0+ bytes
a dataframe is made of Series
. Observe that each series has its own type
s1 = df["Uso_Internet"]
type(s1)
pandas.core.series.Series
s1
Pais
Argentina 49.40
Australia 80.60
Belgium 67.30
Brazil 37.76
Canada 72.30
Chile 50.90
China 22.40
Colombia 38.80
Egypt 12.90
France 65.70
Germany 67.00
Hong_Kong 69.50
India 7.10
Indonesia 10.50
Italy 48.80
Japan 73.80
Malaysia 62.80
Mexico 24.90
Netherlands 82.90
Peru 26.20
Philippines 21.50
Poland 52.00
Russia 27.00
Saudi_Arabia 22.70
South_Africa 10.50
Spain 66.80
Sweden 80.70
Taiwan 66.10
Thailand 20.50
Turkey 35.00
USA 77.33
UK 70.18
Venezuela 25.50
Name: Uso_Internet, dtype: float64
if the column name is not too fancy (empy spaces, accents, etc.) we can use columns names as python syntax.
df.Uso_Facebook
Pais
Argentina 30.53
Australia 46.01
Belgium 36.98
Brazil 4.39
Canada 52.08
Chile 46.14
China 0.05
Colombia 25.90
Egypt 5.68
France 32.91
Germany 14.07
Hong_Kong 52.33
India 1.52
Indonesia 13.49
Italy 30.62
Japan 2.00
Malaysia 37.77
Mexico 16.80
Netherlands 20.54
Peru 13.34
Philippines 19.68
Poland 11.79
Russia 2.99
Saudi_Arabia 11.65
South_Africa 7.83
Spain 30.24
Sweden 44.72
Taiwan 38.21
Thailand 10.29
Turkey 31.91
USA 46.98
UK 45.97
Venezuela 28.64
Name: Uso_Facebook, dtype: float64
DataFrame indexing¶
is NOT exactly like numpy
first index
if string refers to columns
if
Series
of booleans is used as a filter
for selecting columns:
use
.loc
to select by Indexuse
.iloc
to select by position
df["Colombia"]
---------------------------------------------------------------------
KeyError Traceback (most recent call last)
/opt/anaconda3/envs/p37/lib/python3.7/site-packages/pandas/core/indexes/base.py in get_loc(self, key, method, tolerance)
2896 try:
-> 2897 return self._engine.get_loc(key)
2898 except KeyError:
pandas/_libs/index.pyx in pandas._libs.index.IndexEngine.get_loc()
pandas/_libs/index.pyx in pandas._libs.index.IndexEngine.get_loc()
pandas/_libs/hashtable_class_helper.pxi in pandas._libs.hashtable.PyObjectHashTable.get_item()
pandas/_libs/hashtable_class_helper.pxi in pandas._libs.hashtable.PyObjectHashTable.get_item()
KeyError: 'Colombia'
During handling of the above exception, another exception occurred:
KeyError Traceback (most recent call last)
<ipython-input-251-5f701fabe22b> in <module>
----> 1 df["Colombia"]
/opt/anaconda3/envs/p37/lib/python3.7/site-packages/pandas/core/frame.py in __getitem__(self, key)
2993 if self.columns.nlevels > 1:
2994 return self._getitem_multilevel(key)
-> 2995 indexer = self.columns.get_loc(key)
2996 if is_integer(indexer):
2997 indexer = [indexer]
/opt/anaconda3/envs/p37/lib/python3.7/site-packages/pandas/core/indexes/base.py in get_loc(self, key, method, tolerance)
2897 return self._engine.get_loc(key)
2898 except KeyError:
-> 2899 return self._engine.get_loc(self._maybe_cast_indexer(key))
2900 indexer = self.get_indexer([key], method=method, tolerance=tolerance)
2901 if indexer.ndim > 1 or indexer.size > 1:
pandas/_libs/index.pyx in pandas._libs.index.IndexEngine.get_loc()
pandas/_libs/index.pyx in pandas._libs.index.IndexEngine.get_loc()
pandas/_libs/hashtable_class_helper.pxi in pandas._libs.hashtable.PyObjectHashTable.get_item()
pandas/_libs/hashtable_class_helper.pxi in pandas._libs.hashtable.PyObjectHashTable.get_item()
KeyError: 'Colombia'
df.loc["Colombia"]
Uso_Internet 38.8
Uso_Facebook 25.9
Name: Colombia, dtype: float64
Index semantics is exact!!
df.loc["Colombia":"Spain"]
Uso_Internet | Uso_Facebook | |
---|---|---|
Pais | ||
Colombia | 38.8 | 25.90 |
Egypt | 12.9 | 5.68 |
France | 65.7 | 32.91 |
Germany | 67.0 | 14.07 |
Hong_Kong | 69.5 | 52.33 |
India | 7.1 | 1.52 |
Indonesia | 10.5 | 13.49 |
Italy | 48.8 | 30.62 |
Japan | 73.8 | 2.00 |
Malaysia | 62.8 | 37.77 |
Mexico | 24.9 | 16.80 |
Netherlands | 82.9 | 20.54 |
Peru | 26.2 | 13.34 |
Philippines | 21.5 | 19.68 |
Poland | 52.0 | 11.79 |
Russia | 27.0 | 2.99 |
Saudi_Arabia | 22.7 | 11.65 |
South_Africa | 10.5 | 7.83 |
Spain | 66.8 | 30.24 |
df.iloc[10:15]
Uso_Internet | Uso_Facebook | |
---|---|---|
Pais | ||
Germany | 67.0 | 14.07 |
Hong_Kong | 69.5 | 52.33 |
India | 7.1 | 1.52 |
Indonesia | 10.5 | 13.49 |
Italy | 48.8 | 30.62 |
filtering
df[df.Uso_Internet>80]
Uso_Internet | Uso_Facebook | |
---|---|---|
Pais | ||
Australia | 80.6 | 46.01 |
Netherlands | 82.9 | 20.54 |
Sweden | 80.7 | 44.72 |
combined conditions
df[(df.Uso_Internet>50)&(df.Uso_Facebook>50)]
Uso_Internet | Uso_Facebook | |
---|---|---|
Pais | ||
Canada | 72.3 | 52.08 |
Hong_Kong | 69.5 | 52.33 |
df[(df.Uso_Internet>50)|(df.Uso_Facebook>50)]
Uso_Internet | Uso_Facebook | |
---|---|---|
Pais | ||
Australia | 80.60 | 46.01 |
Belgium | 67.30 | 36.98 |
Canada | 72.30 | 52.08 |
Chile | 50.90 | 46.14 |
France | 65.70 | 32.91 |
Germany | 67.00 | 14.07 |
Hong_Kong | 69.50 | 52.33 |
Japan | 73.80 | 2.00 |
Malaysia | 62.80 | 37.77 |
Netherlands | 82.90 | 20.54 |
Poland | 52.00 | 11.79 |
Spain | 66.80 | 30.24 |
Sweden | 80.70 | 44.72 |
Taiwan | 66.10 | 38.21 |
USA | 77.33 | 46.98 |
UK | 70.18 | 45.97 |
Managing data¶
observe csv structure:
missing column name
missing data
!head local/data/comptagevelo2009.csv
Date,,Berri1,Maisonneuve_1,Maisonneuve_2,Brébeuf
01/01/2009,00:00,29,20,35,
02/01/2009,00:00,19,3,22,
03/01/2009,00:00,24,12,22,
04/01/2009,00:00,24,8,15,
05/01/2009,00:00,120,111,141,
06/01/2009,00:00,261,146,236,
07/01/2009,00:00,60,33,80,
08/01/2009,00:00,24,14,14,
09/01/2009,00:00,35,20,32,
d = pd.read_csv("local/data/comptagevelo2009.csv")
d
Date | Unnamed: 1 | Berri1 | Maisonneuve_1 | Maisonneuve_2 | Brébeuf | |
---|---|---|---|---|---|---|
0 | 01/01/2009 | 00:00 | 29 | 20 | 35 | NaN |
1 | 02/01/2009 | 00:00 | 19 | 3 | 22 | NaN |
2 | 03/01/2009 | 00:00 | 24 | 12 | 22 | NaN |
3 | 04/01/2009 | 00:00 | 24 | 8 | 15 | NaN |
4 | 05/01/2009 | 00:00 | 120 | 111 | 141 | NaN |
... | ... | ... | ... | ... | ... | ... |
360 | 27/12/2009 | 00:00 | 66 | 29 | 52 | 0.0 |
361 | 28/12/2009 | 00:00 | 61 | 41 | 99 | 0.0 |
362 | 29/12/2009 | 00:00 | 89 | 52 | 115 | 0.0 |
363 | 30/12/2009 | 00:00 | 76 | 43 | 115 | 0.0 |
364 | 31/12/2009 | 00:00 | 53 | 46 | 112 | 0.0 |
365 rows × 6 columns
d.columns, d.shape
(Index(['Date', 'Unnamed: 1', 'Berri1', 'Maisonneuve_1', 'Maisonneuve_2',
'Brébeuf'],
dtype='object'), (365, 6))
numerical features
d.describe()
Berri1 | Maisonneuve_1 | Maisonneuve_2 | Brébeuf | |
---|---|---|---|---|
count | 365.000000 | 365.000000 | 365.000000 | 178.000000 |
mean | 2032.200000 | 1060.252055 | 2093.169863 | 2576.359551 |
std | 1878.879799 | 1079.533086 | 1854.368523 | 2484.004743 |
min | 0.000000 | 0.000000 | 0.000000 | 0.000000 |
25% | 194.000000 | 90.000000 | 228.000000 | 0.000000 |
50% | 1726.000000 | 678.000000 | 1686.000000 | 1443.500000 |
75% | 3540.000000 | 1882.000000 | 3520.000000 | 4638.000000 |
max | 6626.000000 | 4242.000000 | 6587.000000 | 7575.000000 |
d["Berri1"].head()
0 29
1 19
2 24
3 24
4 120
Name: Berri1, dtype: int64
d["Unnamed: 1"].unique()
array(['00:00'], dtype=object)
d["Berri1"].unique()
array([ 29, 19, 24, 120, 261, 60, 35, 81, 318, 105, 168,
145, 131, 93, 25, 52, 136, 147, 109, 172, 148, 15,
209, 92, 110, 14, 158, 179, 122, 95, 185, 82, 190,
228, 306, 188, 98, 139, 258, 304, 326, 134, 125, 96,
65, 123, 129, 154, 239, 198, 32, 67, 157, 164, 300,
176, 195, 310, 7, 366, 234, 132, 203, 298, 541, 525,
871, 592, 455, 446, 441, 266, 189, 343, 292, 355, 245,
0, 445, 1286, 1178, 2131, 2709, 752, 1886, 2069, 3132, 3668,
1368, 4051, 2286, 3519, 3520, 1925, 2125, 2662, 4403, 4338, 2757,
970, 2767, 1493, 728, 3982, 4742, 5278, 2344, 4094, 784, 1048,
2442, 3686, 3042, 5728, 3815, 3540, 4775, 4434, 4363, 2075, 2338,
1387, 2063, 2031, 3274, 4325, 5430, 6028, 3876, 2742, 4973, 1125,
3460, 4449, 3576, 4027, 4313, 3182, 5668, 6320, 2397, 2857, 2590,
3234, 5138, 5799, 4911, 4333, 3680, 1536, 3064, 1004, 4709, 4471,
4432, 2997, 2544, 5121, 3862, 3036, 3744, 6626, 6274, 1876, 4393,
3471, 3537, 6100, 3489, 4859, 2991, 3588, 5607, 5754, 3440, 5124,
4054, 4372, 1801, 4088, 5891, 3754, 5267, 3146, 63, 77, 5904,
4417, 5611, 4197, 4265, 4589, 2775, 2999, 3504, 5538, 5386, 3916,
3307, 4382, 5327, 3796, 2832, 3492, 2888, 4120, 5450, 4722, 4707,
4439, 2277, 4572, 5298, 5451, 5372, 4566, 3533, 3888, 3683, 5452,
5575, 5496, 4864, 3985, 2695, 4196, 5169, 4891, 4915, 2435, 2674,
2855, 4787, 2620, 2878, 4820, 3774, 2603, 725, 1941, 2272, 3003,
2643, 2865, 993, 1336, 2935, 3852, 2115, 3336, 1302, 1407, 1090,
1171, 1671, 2456, 2383, 1130, 1241, 2570, 2605, 2904, 1322, 1792,
542, 1124, 2119, 2072, 1996, 2130, 1835, 473, 1141, 2293, 1655,
1974, 1767, 1735, 872, 1541, 2540, 2526, 2366, 2224, 2007, 493,
852, 1881, 2052, 1921, 1935, 1065, 1173, 743, 1579, 1574, 1726,
1027, 810, 671, 747, 1092, 1377, 606, 1108, 594, 501, 669,
570, 219, 194, 106, 130, 271, 308, 296, 214, 133, 135,
207, 74, 34, 40, 66, 61, 89, 76, 53])
d["Berri1"].dtype, d["Date"].dtype, d["Unnamed: 1"].dtype
(dtype('int64'), dtype('O'), dtype('O'))
d.index
RangeIndex(start=0, stop=365, step=1)
Fixing data¶
observe we set one column as the index one, and we convert it to date object type
d.Date
0 01/01/2009
1 02/01/2009
2 03/01/2009
3 04/01/2009
4 05/01/2009
...
360 27/12/2009
361 28/12/2009
362 29/12/2009
363 30/12/2009
364 31/12/2009
Name: Date, Length: 365, dtype: object
d.index = pd.to_datetime(d.Date)
del(d["Date"])
del(d["Unnamed: 1"])
d.head()
Berri1 | Maisonneuve_1 | Maisonneuve_2 | Brébeuf | |
---|---|---|---|---|
Date | ||||
2009-01-01 | 29 | 20 | 35 | NaN |
2009-02-01 | 19 | 3 | 22 | NaN |
2009-03-01 | 24 | 12 | 22 | NaN |
2009-04-01 | 24 | 8 | 15 | NaN |
2009-05-01 | 120 | 111 | 141 | NaN |
d.index
DatetimeIndex(['2009-01-01', '2009-02-01', '2009-03-01', '2009-04-01',
'2009-05-01', '2009-06-01', '2009-07-01', '2009-08-01',
'2009-09-01', '2009-10-01',
...
'2009-12-22', '2009-12-23', '2009-12-24', '2009-12-25',
'2009-12-26', '2009-12-27', '2009-12-28', '2009-12-29',
'2009-12-30', '2009-12-31'],
dtype='datetime64[ns]', name='Date', length=365, freq=None)
let’s fix columns names
d.columns=["Berri", "Mneuve1", "Mneuve2", "Brebeuf"]
d.head()
Berri | Mneuve1 | Mneuve2 | Brebeuf | |
---|---|---|---|---|
Date | ||||
2009-01-01 | 29 | 20 | 35 | NaN |
2009-02-01 | 19 | 3 | 22 | NaN |
2009-03-01 | 24 | 12 | 22 | NaN |
2009-04-01 | 24 | 8 | 15 | NaN |
2009-05-01 | 120 | 111 | 141 | NaN |
for col in d.columns:
print (col, np.sum(pd.isnull(d[col])))
Berri 0
Mneuve1 0
Mneuve2 0
Brebeuf 187
d.shape
(365, 4)
d['Brebeuf'].describe()
count 178.000000
mean 2576.359551
std 2484.004743
min 0.000000
25% 0.000000
50% 1443.500000
75% 4638.000000
max 7575.000000
Name: Brebeuf, dtype: float64
plt.hist(d.Brebeuf, bins=30);
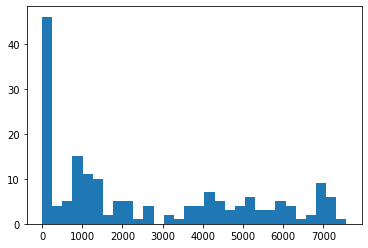
fix missing!!!
d.Brebeuf.fillna(d.Brebeuf.mean(), inplace=True)
d['Brebeuf'].describe()
count 365.000000
mean 2576.359551
std 1732.161423
min 0.000000
25% 1588.000000
50% 2576.359551
75% 2576.359551
max 7575.000000
Name: Brebeuf, dtype: float64
plt.hist(d.Brebeuf, bins=30);
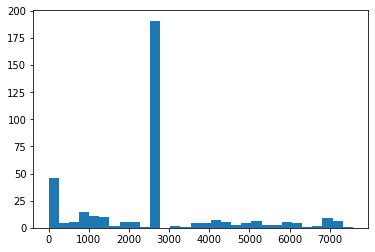
d
Berri | Mneuve1 | Mneuve2 | Brebeuf | |
---|---|---|---|---|
Date | ||||
2009-01-01 | 29 | 20 | 35 | 2576.359551 |
2009-02-01 | 19 | 3 | 22 | 2576.359551 |
2009-03-01 | 24 | 12 | 22 | 2576.359551 |
2009-04-01 | 24 | 8 | 15 | 2576.359551 |
2009-05-01 | 120 | 111 | 141 | 2576.359551 |
... | ... | ... | ... | ... |
2009-12-27 | 66 | 29 | 52 | 0.000000 |
2009-12-28 | 61 | 41 | 99 | 0.000000 |
2009-12-29 | 89 | 52 | 115 | 0.000000 |
2009-12-30 | 76 | 43 | 115 | 0.000000 |
2009-12-31 | 53 | 46 | 112 | 0.000000 |
365 rows × 4 columns
let’s make sure it is sorted
d.sort_index(inplace=True)
d.head()
Berri | Mneuve1 | Mneuve2 | Brebeuf | |
---|---|---|---|---|
Date | ||||
2009-01-01 | 29 | 20 | 35 | 2576.359551 |
2009-01-02 | 14 | 2 | 2 | 2576.359551 |
2009-01-03 | 67 | 30 | 80 | 2576.359551 |
2009-01-04 | 0 | 0 | 0 | 2576.359551 |
2009-01-05 | 1925 | 1256 | 1501 | 2576.359551 |
Filtering¶
d[d.Berri>6000]
Berri | Mneuve1 | Mneuve2 | Brebeuf | |
---|---|---|---|---|
Date | ||||
2009-05-06 | 6028 | 4120 | 4223 | 2576.359551 |
2009-06-17 | 6320 | 3388 | 6047 | 2576.359551 |
2009-07-15 | 6100 | 3767 | 5536 | 6939.000000 |
2009-09-07 | 6626 | 4227 | 5751 | 7575.000000 |
2009-10-07 | 6274 | 4242 | 5435 | 7268.000000 |
d[(d.Berri>6000) & (d.Brebeuf<7000)]
Berri | Mneuve1 | Mneuve2 | Brebeuf | |
---|---|---|---|---|
Date | ||||
2009-05-06 | 6028 | 4120 | 4223 | 2576.359551 |
2009-06-17 | 6320 | 3388 | 6047 | 2576.359551 |
2009-07-15 | 6100 | 3767 | 5536 | 6939.000000 |
Locating¶
d[d.Berri>5500].sort_index(axis=0)
Berri | Mneuve1 | Mneuve2 | Brebeuf | |
---|---|---|---|---|
Date | ||||
2009-03-08 | 5904 | 3102 | 4853 | 7194.000000 |
2009-05-06 | 6028 | 4120 | 4223 | 2576.359551 |
2009-05-08 | 5611 | 2646 | 5201 | 7121.000000 |
2009-05-21 | 5728 | 3693 | 5397 | 2576.359551 |
2009-06-16 | 5668 | 3499 | 5609 | 2576.359551 |
2009-06-17 | 6320 | 3388 | 6047 | 2576.359551 |
2009-06-23 | 5799 | 3114 | 5386 | 2576.359551 |
2009-07-15 | 6100 | 3767 | 5536 | 6939.000000 |
2009-07-20 | 5607 | 3825 | 5092 | 7064.000000 |
2009-07-21 | 5754 | 3745 | 5357 | 6996.000000 |
2009-07-28 | 5891 | 3292 | 5437 | 7219.000000 |
2009-09-07 | 6626 | 4227 | 5751 | 7575.000000 |
2009-09-09 | 5575 | 2727 | 6535 | 6686.000000 |
2009-10-07 | 6274 | 4242 | 5435 | 7268.000000 |
2009-12-08 | 5538 | 2368 | 5107 | 7127.000000 |
d.iloc[100:110]
Berri | Mneuve1 | Mneuve2 | Brebeuf | |
---|---|---|---|---|
Date | ||||
2009-04-11 | 1974 | 1113 | 2693 | 1046.000000 |
2009-04-12 | 1108 | 595 | 1472 | 0.000000 |
2009-04-13 | 0 | 0 | 0 | 2576.359551 |
2009-04-14 | 0 | 0 | 0 | 2576.359551 |
2009-04-15 | 0 | 0 | 0 | 2576.359551 |
2009-04-16 | 0 | 0 | 0 | 2576.359551 |
2009-04-17 | 1286 | 820 | 1436 | 2576.359551 |
2009-04-18 | 1178 | 667 | 826 | 2576.359551 |
2009-04-19 | 2131 | 1155 | 1426 | 2576.359551 |
2009-04-20 | 2709 | 1697 | 2646 | 2576.359551 |
dates as INDEX have special semantics
d.loc["2009-10-01":"2009-10-10"]
Berri | Mneuve1 | Mneuve2 | Brebeuf | |
---|---|---|---|---|
Date | ||||
2009-10-01 | 81 | 45 | 79 | 2576.359551 |
2009-10-02 | 228 | 101 | 260 | 2576.359551 |
2009-10-03 | 366 | 203 | 354 | 2576.359551 |
2009-10-04 | 0 | 0 | 0 | 2576.359551 |
2009-10-05 | 728 | 362 | 523 | 2576.359551 |
2009-10-06 | 3460 | 2354 | 3978 | 2576.359551 |
2009-10-07 | 6274 | 4242 | 5435 | 7268.000000 |
2009-10-08 | 2999 | 1545 | 3185 | 4187.000000 |
2009-10-09 | 5496 | 2921 | 6587 | 6520.000000 |
2009-10-10 | 1407 | 725 | 1443 | 1003.000000 |
can do sorting across any criteria
d.sort_values(by="Berri").head()
Berri | Mneuve1 | Mneuve2 | Brebeuf | |
---|---|---|---|---|
Date | ||||
2009-07-04 | 0 | 0 | 0 | 2576.359551 |
2009-03-30 | 0 | 0 | 0 | 2576.359551 |
2009-04-04 | 0 | 0 | 0 | 2576.359551 |
2009-04-13 | 0 | 0 | 0 | 2576.359551 |
2009-04-14 | 0 | 0 | 0 | 2576.359551 |
and chain operations
d.sort_values(by="Berri").loc["2009-10-01":"2009-10-10"]
Berri | Mneuve1 | Mneuve2 | Brebeuf | |
---|---|---|---|---|
Date | ||||
2009-10-04 | 0 | 0 | 0 | 2576.359551 |
2009-10-01 | 81 | 45 | 79 | 2576.359551 |
2009-10-02 | 228 | 101 | 260 | 2576.359551 |
2009-10-03 | 366 | 203 | 354 | 2576.359551 |
2009-10-05 | 728 | 362 | 523 | 2576.359551 |
2009-10-10 | 1407 | 725 | 1443 | 1003.000000 |
2009-10-08 | 2999 | 1545 | 3185 | 4187.000000 |
2009-10-06 | 3460 | 2354 | 3978 | 2576.359551 |
2009-10-09 | 5496 | 2921 | 6587 | 6520.000000 |
2009-10-07 | 6274 | 4242 | 5435 | 7268.000000 |
Time series operations¶
d.rolling(3).mean().head(10)
Berri | Mneuve1 | Mneuve2 | Brebeuf | |
---|---|---|---|---|
Date | ||||
2009-01-01 | NaN | NaN | NaN | NaN |
2009-01-02 | NaN | NaN | NaN | NaN |
2009-01-03 | 36.666667 | 17.333333 | 39.000000 | 2576.359551 |
2009-01-04 | 27.000000 | 10.666667 | 27.333333 | 2576.359551 |
2009-01-05 | 664.000000 | 428.666667 | 527.000000 | 2576.359551 |
2009-01-06 | 1733.000000 | 1116.333333 | 1409.000000 | 2576.359551 |
2009-01-07 | 3223.333333 | 1862.666667 | 2426.000000 | 2576.359551 |
2009-01-08 | 2602.666667 | 1795.000000 | 3042.666667 | 3673.906367 |
2009-01-09 | 3277.333333 | 2029.333333 | 4055.666667 | 5128.119850 |
2009-01-10 | 2668.000000 | 1290.333333 | 4214.666667 | 4798.666667 |
d.index = d.index + pd.Timedelta("5m")
d.head()
Berri | Mneuve1 | Mneuve2 | Brebeuf | |
---|---|---|---|---|
Date | ||||
2009-01-01 00:05:00 | 29 | 20 | 35 | 2576.359551 |
2009-01-02 00:05:00 | 14 | 2 | 2 | 2576.359551 |
2009-01-03 00:05:00 | 67 | 30 | 80 | 2576.359551 |
2009-01-04 00:05:00 | 0 | 0 | 0 | 2576.359551 |
2009-01-05 00:05:00 | 1925 | 1256 | 1501 | 2576.359551 |
d.shift(freq=pd.Timedelta(days=365)).head()
Berri | Mneuve1 | Mneuve2 | Brebeuf | |
---|---|---|---|---|
Date | ||||
2010-01-01 00:05:00 | 29 | 20 | 35 | 2576.359551 |
2010-01-02 00:05:00 | 14 | 2 | 2 | 2576.359551 |
2010-01-03 00:05:00 | 67 | 30 | 80 | 2576.359551 |
2010-01-04 00:05:00 | 0 | 0 | 0 | 2576.359551 |
2010-01-05 00:05:00 | 1925 | 1256 | 1501 | 2576.359551 |
Downsampling¶
d.resample(pd.Timedelta("2d")).first().head()
Berri | Mneuve1 | Mneuve2 | Brebeuf | |
---|---|---|---|---|
Date | ||||
2009-01-01 | 29 | 20 | 35 | 2576.359551 |
2009-01-03 | 67 | 30 | 80 | 2576.359551 |
2009-01-05 | 1925 | 1256 | 1501 | 2576.359551 |
2009-01-07 | 4471 | 2239 | 3051 | 2576.359551 |
2009-01-09 | 5298 | 2796 | 5765 | 6939.000000 |
d.resample(pd.Timedelta("2d")).mean().head()
Berri | Mneuve1 | Mneuve2 | Brebeuf | |
---|---|---|---|---|
Date | ||||
2009-01-01 | 21.5 | 11.0 | 18.5 | 2576.359551 |
2009-01-03 | 33.5 | 15.0 | 40.0 | 2576.359551 |
2009-01-05 | 2599.5 | 1674.5 | 2113.5 | 2576.359551 |
2009-01-07 | 2267.0 | 1646.0 | 3201.0 | 4222.679775 |
2009-01-09 | 3970.5 | 1409.0 | 4646.5 | 4263.500000 |
Upsampling¶
d.resample(pd.Timedelta("12h")).first().head()
Berri | Mneuve1 | Mneuve2 | Brebeuf | |
---|---|---|---|---|
Date | ||||
2009-01-01 00:00:00 | 29.0 | 20.0 | 35.0 | 2576.359551 |
2009-01-01 12:00:00 | NaN | NaN | NaN | NaN |
2009-01-02 00:00:00 | 14.0 | 2.0 | 2.0 | 2576.359551 |
2009-01-02 12:00:00 | NaN | NaN | NaN | NaN |
2009-01-03 00:00:00 | 67.0 | 30.0 | 80.0 | 2576.359551 |
d.resample(pd.Timedelta("12h")).fillna(method="pad").head()
Berri | Mneuve1 | Mneuve2 | Brebeuf | |
---|---|---|---|---|
Date | ||||
2009-01-01 00:00:00 | NaN | NaN | NaN | NaN |
2009-01-01 12:00:00 | 29.0 | 20.0 | 35.0 | 2576.359551 |
2009-01-02 00:00:00 | 29.0 | 20.0 | 35.0 | 2576.359551 |
2009-01-02 12:00:00 | 14.0 | 2.0 | 2.0 | 2576.359551 |
2009-01-03 00:00:00 | 14.0 | 2.0 | 2.0 | 2576.359551 |
Building Dataframes from other structures¶
a = np.random.randint(10,size=(20,5))
a
array([[6, 4, 8, 2, 0],
[8, 9, 7, 4, 9],
[3, 3, 5, 0, 2],
[8, 9, 3, 9, 1],
[6, 9, 4, 5, 9],
[8, 6, 1, 0, 1],
[9, 6, 1, 7, 2],
[0, 7, 4, 6, 1],
[2, 2, 5, 3, 7],
[4, 9, 3, 3, 9],
[7, 3, 5, 6, 8],
[6, 7, 6, 2, 8],
[1, 2, 5, 2, 8],
[6, 7, 6, 9, 4],
[9, 4, 5, 5, 1],
[4, 4, 5, 9, 8],
[4, 4, 1, 1, 2],
[2, 8, 9, 7, 7],
[3, 4, 3, 0, 5],
[5, 8, 5, 6, 2]])
k = pd.DataFrame(a, columns=["uno", "dos", "tres", "cuatro", "cinco"], index=range(10,10+len(a)))
k
uno | dos | tres | cuatro | cinco | |
---|---|---|---|---|---|
10 | 6 | 4 | 8 | 2 | 0 |
11 | 8 | 9 | 7 | 4 | 9 |
12 | 3 | 3 | 5 | 0 | 2 |
13 | 8 | 9 | 3 | 9 | 1 |
14 | 6 | 9 | 4 | 5 | 9 |
15 | 8 | 6 | 1 | 0 | 1 |
16 | 9 | 6 | 1 | 7 | 2 |
17 | 0 | 7 | 4 | 6 | 1 |
18 | 2 | 2 | 5 | 3 | 7 |
19 | 4 | 9 | 3 | 3 | 9 |
20 | 7 | 3 | 5 | 6 | 8 |
21 | 6 | 7 | 6 | 2 | 8 |
22 | 1 | 2 | 5 | 2 | 8 |
23 | 6 | 7 | 6 | 9 | 4 |
24 | 9 | 4 | 5 | 5 | 1 |
25 | 4 | 4 | 5 | 9 | 8 |
26 | 4 | 4 | 1 | 1 | 2 |
27 | 2 | 8 | 9 | 7 | 7 |
28 | 3 | 4 | 3 | 0 | 5 |
29 | 5 | 8 | 5 | 6 | 2 |
.values
access the underlying numpy
structure¶
d.values
array([[2.90000000e+01, 2.00000000e+01, 3.50000000e+01, 2.57635955e+03],
[1.40000000e+01, 2.00000000e+00, 2.00000000e+00, 2.57635955e+03],
[6.70000000e+01, 3.00000000e+01, 8.00000000e+01, 2.57635955e+03],
...,
[8.90000000e+01, 5.20000000e+01, 1.15000000e+02, 0.00000000e+00],
[7.60000000e+01, 4.30000000e+01, 1.15000000e+02, 0.00000000e+00],
[5.30000000e+01, 4.60000000e+01, 1.12000000e+02, 0.00000000e+00]])
some out-of-the-box plotting¶
but recall that we always can do custom plotting
d.plot(figsize=(15,3))
<matplotlib.axes._subplots.AxesSubplot at 0x7fb9f2a9ac10>
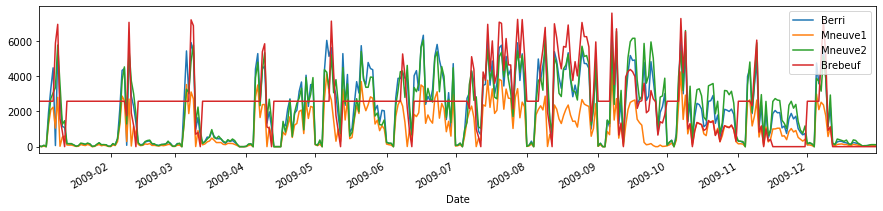
plt.figure(figsize=(15,3))
plt.plot(d.Berri)
[<matplotlib.lines.Line2D at 0x7fb9f29f2250>]
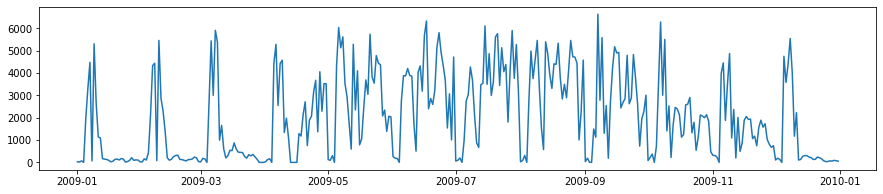
d.Berri.cumsum().plot()
<matplotlib.axes._subplots.AxesSubplot at 0x7fb9f294c450>
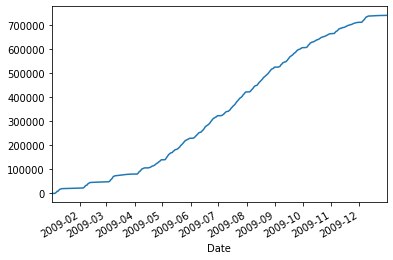
plt.scatter(d.Berri, d.Brebeuf)
<matplotlib.collections.PathCollection at 0x7fb9f360fb50>
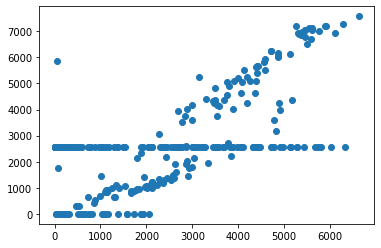
pd.plotting.scatter_matrix(d, figsize=(10,10));
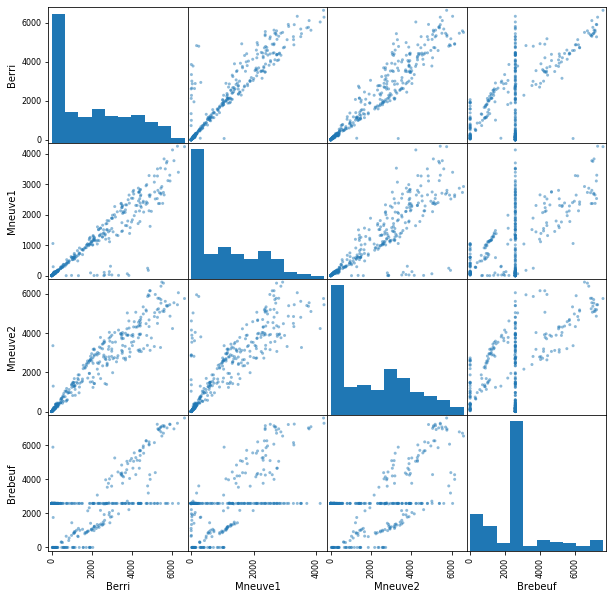
Grouping¶
d["month"] = [i.month for i in d.index]
d.head()
Berri | Mneuve1 | Mneuve2 | Brebeuf | month | |
---|---|---|---|---|---|
Date | |||||
2009-01-01 00:05:00 | 29 | 20 | 35 | 2576.359551 | 1 |
2009-01-02 00:05:00 | 14 | 2 | 2 | 2576.359551 | 1 |
2009-01-03 00:05:00 | 67 | 30 | 80 | 2576.359551 | 1 |
2009-01-04 00:05:00 | 0 | 0 | 0 | 2576.359551 | 1 |
2009-01-05 00:05:00 | 1925 | 1256 | 1501 | 2576.359551 | 1 |
d.groupby("month").max()
Berri | Mneuve1 | Mneuve2 | Brebeuf | |
---|---|---|---|---|
month | ||||
1 | 5298 | 2796 | 5765 | 6939.0 |
2 | 5451 | 2868 | 5517 | 7052.0 |
3 | 5904 | 3523 | 5762 | 7194.0 |
4 | 5278 | 3499 | 5327 | 5837.0 |
5 | 6028 | 4120 | 5397 | 7121.0 |
6 | 6320 | 3499 | 6047 | 5259.0 |
7 | 6100 | 3825 | 5536 | 7219.0 |
8 | 5452 | 2865 | 6379 | 7044.0 |
9 | 6626 | 4227 | 6535 | 7575.0 |
10 | 6274 | 4242 | 6587 | 7268.0 |
11 | 4864 | 2648 | 5895 | 6044.0 |
12 | 5538 | 2983 | 5107 | 7127.0 |
d.groupby("month").count()
Berri | Mneuve1 | Mneuve2 | Brebeuf | |
---|---|---|---|---|
month | ||||
1 | 31 | 31 | 31 | 31 |
2 | 28 | 28 | 28 | 28 |
3 | 31 | 31 | 31 | 31 |
4 | 30 | 30 | 30 | 30 |
5 | 31 | 31 | 31 | 31 |
6 | 30 | 30 | 30 | 30 |
7 | 31 | 31 | 31 | 31 |
8 | 31 | 31 | 31 | 31 |
9 | 30 | 30 | 30 | 30 |
10 | 31 | 31 | 31 | 31 |
11 | 30 | 30 | 30 | 30 |
12 | 31 | 31 | 31 | 31 |
Time series¶
observe we can establish at load time many thing if the dataset is relatively clean
tiempo=pd.read_csv('local/data/weather_data_austin_2010.csv',parse_dates=['Date'], dayfirst=True ,index_col='Date')
tiempo
Temperature | DewPoint | Pressure | |
---|---|---|---|
Date | |||
2010-01-01 00:00:00 | 46.2 | 37.5 | 1.0 |
2010-01-01 01:00:00 | 44.6 | 37.1 | 1.0 |
2010-01-01 02:00:00 | 44.1 | 36.9 | 1.0 |
2010-01-01 03:00:00 | 43.8 | 36.9 | 1.0 |
2010-01-01 04:00:00 | 43.5 | 36.8 | 1.0 |
... | ... | ... | ... |
2010-12-31 19:00:00 | 51.1 | 38.1 | 1.0 |
2010-12-31 20:00:00 | 49.0 | 37.9 | 1.0 |
2010-12-31 21:00:00 | 47.9 | 37.9 | 1.0 |
2010-12-31 22:00:00 | 46.9 | 37.9 | 1.0 |
2010-12-31 23:00:00 | 46.2 | 37.7 | 1.0 |
8759 rows × 3 columns
tiempo.loc['2010-08-01':'2010-10-30']
Temperature | DewPoint | Pressure | |
---|---|---|---|
Date | |||
2010-08-01 00:00:00 | 79.0 | 70.8 | 1.0 |
2010-08-01 01:00:00 | 77.4 | 71.2 | 1.0 |
2010-08-01 02:00:00 | 76.4 | 71.3 | 1.0 |
2010-08-01 03:00:00 | 75.7 | 71.4 | 1.0 |
2010-08-01 04:00:00 | 75.1 | 71.4 | 1.0 |
... | ... | ... | ... |
2010-10-30 19:00:00 | 65.4 | 53.6 | 1.0 |
2010-10-30 20:00:00 | 63.6 | 53.9 | 1.0 |
2010-10-30 21:00:00 | 62.2 | 53.8 | 1.0 |
2010-10-30 22:00:00 | 61.4 | 54.0 | 1.0 |
2010-10-30 23:00:00 | 60.3 | 53.9 | 1.0 |
2184 rows × 3 columns
tiempo.loc['2010-06'].head()
Temperature | DewPoint | Pressure | |
---|---|---|---|
Date | |||
2010-06-01 00:00:00 | 74.0 | 67.9 | 1.0 |
2010-06-01 01:00:00 | 72.6 | 68.0 | 1.0 |
2010-06-01 02:00:00 | 72.0 | 67.9 | 1.0 |
2010-06-01 03:00:00 | 71.6 | 67.9 | 1.0 |
2010-06-01 04:00:00 | 71.1 | 67.7 | 1.0 |
tiempo.sample(10)
Temperature | DewPoint | Pressure | |
---|---|---|---|
Date | |||
2010-12-24 20:00:00 | 48.9 | 38.6 | 1.0 |
2010-10-12 01:00:00 | 64.7 | 59.3 | 1.0 |
2010-02-21 03:00:00 | 49.3 | 42.8 | 1.0 |
2010-07-20 16:00:00 | 93.5 | 67.1 | 1.0 |
2010-11-04 12:00:00 | 70.4 | 53.0 | 1.0 |
2010-10-29 15:00:00 | 75.4 | 53.0 | 1.0 |
2010-07-18 17:00:00 | 92.5 | 67.3 | 1.0 |
2010-04-16 13:00:00 | 76.4 | 56.7 | 1.0 |
2010-07-05 06:00:00 | 74.4 | 71.2 | 1.0 |
2010-07-03 16:00:00 | 91.4 | 68.6 | 1.0 |
tiempo.sample(frac=0.01)
Temperature | DewPoint | Pressure | |
---|---|---|---|
Date | |||
2010-12-22 23:00:00 | 45.9 | 37.8 | 1.0 |
2010-05-19 15:00:00 | 84.9 | 64.8 | 1.0 |
2010-02-24 12:00:00 | 61.9 | 44.5 | 1.0 |
2010-01-10 00:00:00 | 46.2 | 37.4 | 1.0 |
2010-12-21 19:00:00 | 50.7 | 38.8 | 1.0 |
... | ... | ... | ... |
2010-05-27 11:00:00 | 81.7 | 67.3 | 1.0 |
2010-08-11 03:00:00 | 75.8 | 71.3 | 1.0 |
2010-03-17 13:00:00 | 68.5 | 49.6 | 1.0 |
2010-10-28 14:00:00 | 75.2 | 53.9 | 1.0 |
2010-08-21 03:00:00 | 75.9 | 71.1 | 1.0 |
88 rows × 3 columns
Resampling¶
tiempo.head()
Temperature | DewPoint | Pressure | |
---|---|---|---|
Date | |||
2010-01-01 00:00:00 | 46.2 | 37.5 | 1.0 |
2010-01-01 01:00:00 | 44.6 | 37.1 | 1.0 |
2010-01-01 02:00:00 | 44.1 | 36.9 | 1.0 |
2010-01-01 03:00:00 | 43.8 | 36.9 | 1.0 |
2010-01-01 04:00:00 | 43.5 | 36.8 | 1.0 |
tiempo.resample("5d").mean().head()
Temperature | DewPoint | Pressure | |
---|---|---|---|
Date | |||
2010-01-01 | 49.720833 | 38.092500 | 1.0 |
2010-01-06 | 49.449167 | 37.575000 | 1.0 |
2010-01-11 | 49.222500 | 37.603333 | 1.0 |
2010-01-16 | 49.441667 | 37.650000 | 1.0 |
2010-01-21 | 50.683333 | 39.309167 | 1.0 |
tiempo.resample("5d").mean().head()
Temperature | DewPoint | Pressure | |
---|---|---|---|
Date | |||
2010-01-01 | 49.720833 | 38.092500 | 1.0 |
2010-01-06 | 49.449167 | 37.575000 | 1.0 |
2010-01-11 | 49.222500 | 37.603333 | 1.0 |
2010-01-16 | 49.441667 | 37.650000 | 1.0 |
2010-01-21 | 50.683333 | 39.309167 | 1.0 |
tiempo.resample("5d").mean().head()
Temperature | DewPoint | Pressure | |
---|---|---|---|
Date | |||
2010-01-01 | 49.720833 | 38.092500 | 1.0 |
2010-01-06 | 49.449167 | 37.575000 | 1.0 |
2010-01-11 | 49.222500 | 37.603333 | 1.0 |
2010-01-16 | 49.441667 | 37.650000 | 1.0 |
2010-01-21 | 50.683333 | 39.309167 | 1.0 |
tiempo.resample("30min").mean()[:15]
Temperature | DewPoint | Pressure | |
---|---|---|---|
Date | |||
2010-01-01 00:00:00 | 46.2 | 37.5 | 1.0 |
2010-01-01 00:30:00 | NaN | NaN | NaN |
2010-01-01 01:00:00 | 44.6 | 37.1 | 1.0 |
2010-01-01 01:30:00 | NaN | NaN | NaN |
2010-01-01 02:00:00 | 44.1 | 36.9 | 1.0 |
2010-01-01 02:30:00 | NaN | NaN | NaN |
2010-01-01 03:00:00 | 43.8 | 36.9 | 1.0 |
2010-01-01 03:30:00 | NaN | NaN | NaN |
2010-01-01 04:00:00 | 43.5 | 36.8 | 1.0 |
2010-01-01 04:30:00 | NaN | NaN | NaN |
2010-01-01 05:00:00 | 43.0 | 36.5 | 1.0 |
2010-01-01 05:30:00 | NaN | NaN | NaN |
2010-01-01 06:00:00 | 43.1 | 36.3 | 1.0 |
2010-01-01 06:30:00 | NaN | NaN | NaN |
2010-01-01 07:00:00 | 42.3 | 35.9 | 1.0 |
subt=tiempo.between_time(start_time='1:00',end_time='12:00')
subt
Temperature | DewPoint | Pressure | |
---|---|---|---|
Date | |||
2010-01-01 01:00:00 | 44.6 | 37.1 | 1.0 |
2010-01-01 02:00:00 | 44.1 | 36.9 | 1.0 |
2010-01-01 03:00:00 | 43.8 | 36.9 | 1.0 |
2010-01-01 04:00:00 | 43.5 | 36.8 | 1.0 |
2010-01-01 05:00:00 | 43.0 | 36.5 | 1.0 |
... | ... | ... | ... |
2010-12-31 08:00:00 | 42.5 | 36.1 | 1.0 |
2010-12-31 09:00:00 | 46.0 | 37.7 | 1.0 |
2010-12-31 10:00:00 | 49.4 | 38.0 | 1.0 |
2010-12-31 11:00:00 | 52.4 | 38.0 | 1.0 |
2010-12-31 12:00:00 | 54.7 | 37.9 | 1.0 |
4379 rows × 3 columns
tiempo.index.weekday
Int64Index([4, 4, 4, 4, 4, 4, 4, 4, 4, 4,
...
4, 4, 4, 4, 4, 4, 4, 4, 4, 4],
dtype='int64', name='Date', length=8759)
tiempo.index.month
Int64Index([ 1, 1, 1, 1, 1, 1, 1, 1, 1, 1,
...
12, 12, 12, 12, 12, 12, 12, 12, 12, 12],
dtype='int64', name='Date', length=8759)
tiempo.index.day
Int64Index([ 1, 1, 1, 1, 1, 1, 1, 1, 1, 1,
...
31, 31, 31, 31, 31, 31, 31, 31, 31, 31],
dtype='int64', name='Date', length=8759)
tiempo.plot(style='.')
<matplotlib.axes._subplots.AxesSubplot at 0x7fb9e70c8250>
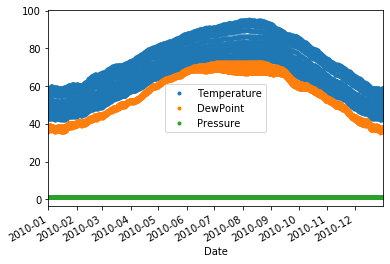
tiempo['2010-01'].plot()
<matplotlib.axes._subplots.AxesSubplot at 0x7fb9f2af66d0>
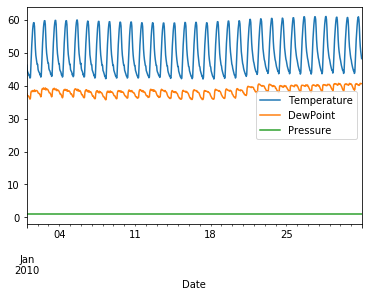
tiempo['2010-01-04'].plot()
<matplotlib.axes._subplots.AxesSubplot at 0x7fb9f2b8eed0>
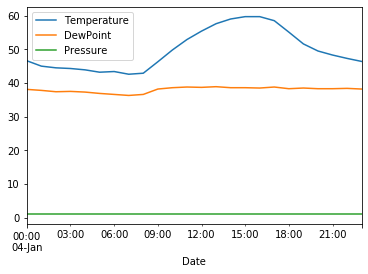
Rolling operations¶
import pandas as pd
### permite obtener data frames directamente de internet
!pip install yfinance
Collecting yfinance
Downloading https://files.pythonhosted.org/packages/c2/31/8b374a12b90def92a4e27d0fc595fc43635f395984e36a075244d98bd265/yfinance-0.1.54.tar.gz
Collecting pandas>=0.24
?25l Downloading https://files.pythonhosted.org/packages/4a/6a/94b219b8ea0f2d580169e85ed1edc0163743f55aaeca8a44c2e8fc1e344e/pandas-1.0.3-cp37-cp37m-manylinux1_x86_64.whl (10.0MB)
|████████████████████████████████| 10.0MB 453kB/s eta 0:00:01
?25hRequirement already satisfied: numpy>=1.15 in /opt/anaconda3/lib/python3.7/site-packages (from yfinance) (1.15.1)
Requirement already satisfied: requests>=2.20 in /opt/anaconda3/lib/python3.7/site-packages (from yfinance) (2.22.0)
Collecting multitasking>=0.0.7
Downloading https://files.pythonhosted.org/packages/69/e7/e9f1661c28f7b87abfa08cb0e8f51dad2240a9f4f741f02ea839835e6d18/multitasking-0.0.9.tar.gz
Requirement already satisfied: pytz>=2017.2 in /opt/anaconda3/lib/python3.7/site-packages (from pandas>=0.24->yfinance) (2018.5)
Requirement already satisfied: python-dateutil>=2.6.1 in /opt/anaconda3/lib/python3.7/site-packages (from pandas>=0.24->yfinance) (2.7.3)
Requirement already satisfied: idna<2.9,>=2.5 in /opt/anaconda3/lib/python3.7/site-packages (from requests>=2.20->yfinance) (2.8)
Requirement already satisfied: certifi>=2017.4.17 in /opt/anaconda3/lib/python3.7/site-packages (from requests>=2.20->yfinance) (2019.9.11)
Requirement already satisfied: chardet<3.1.0,>=3.0.2 in /opt/anaconda3/lib/python3.7/site-packages (from requests>=2.20->yfinance) (3.0.4)
Requirement already satisfied: urllib3!=1.25.0,!=1.25.1,<1.26,>=1.21.1 in /opt/anaconda3/lib/python3.7/site-packages (from requests>=2.20->yfinance) (1.24.2)
Requirement already satisfied: six>=1.5 in /opt/anaconda3/lib/python3.7/site-packages (from python-dateutil>=2.6.1->pandas>=0.24->yfinance) (1.13.0)
Building wheels for collected packages: yfinance, multitasking
Building wheel for yfinance (setup.py) ... ?25ldone
?25h Created wheel for yfinance: filename=yfinance-0.1.54-py2.py3-none-any.whl size=22411 sha256=d937a7a089b0883844df4d4f388c8bcc4dc145446c43e698a5181e6ebd099621
Stored in directory: /home/rlx/.cache/pip/wheels/f9/e3/5b/ec24dd2984b12d61e0abf26289746c2436a0e7844f26f2515c
Building wheel for multitasking (setup.py) ... ?25ldone
?25h Created wheel for multitasking: filename=multitasking-0.0.9-cp37-none-any.whl size=8368 sha256=bfe866419b7d2ac5e39bab8a20d2378ac727b56d6dc6e3f248e2a4115ea368bb
Stored in directory: /home/rlx/.cache/pip/wheels/37/fa/73/d492849e319038eb4d986f5152e4b19ffb1bc0639da84d2677
Successfully built yfinance multitasking
Installing collected packages: pandas, multitasking, yfinance
Found existing installation: pandas 0.23.4
Uninstalling pandas-0.23.4:
Successfully uninstalled pandas-0.23.4
Successfully installed multitasking-0.0.9 pandas-1.0.3 yfinance-0.1.54
import yfinance as yf
#define the ticker symbol
tickerSymbol = 'MSFT'
#get data on this ticker
tickerData = yf.Ticker(tickerSymbol)
#get the historical prices for this ticker
gs = tickerData.history(period='1d', start='2010-1-1', end='2020-1-25')
#see your data
gs
Open | High | Low | Close | Volume | Dividends | Stock Splits | |
---|---|---|---|---|---|---|---|
Date | |||||||
2010-01-04 | 24.04 | 24.41 | 24.01 | 24.29 | 38409100 | 0.0 | 0 |
2010-01-05 | 24.22 | 24.41 | 24.05 | 24.30 | 49749600 | 0.0 | 0 |
2010-01-06 | 24.24 | 24.40 | 23.96 | 24.15 | 58182400 | 0.0 | 0 |
2010-01-07 | 24.04 | 24.10 | 23.70 | 23.90 | 50559700 | 0.0 | 0 |
2010-01-08 | 23.77 | 24.24 | 23.74 | 24.07 | 51197400 | 0.0 | 0 |
... | ... | ... | ... | ... | ... | ... | ... |
2020-01-17 | 166.96 | 167.01 | 164.98 | 166.64 | 34371700 | 0.0 | 0 |
2020-01-21 | 166.23 | 167.73 | 165.98 | 166.05 | 29517200 | 0.0 | 0 |
2020-01-22 | 166.94 | 167.03 | 165.23 | 165.25 | 24138800 | 0.0 | 0 |
2020-01-23 | 165.74 | 166.35 | 164.82 | 166.27 | 19680800 | 0.0 | 0 |
2020-01-24 | 167.05 | 167.07 | 164.00 | 164.59 | 24918100 | 0.0 | 0 |
2532 rows × 7 columns
gs.Close.rolling(10).mean().head(20)
Date
2010-01-04 NaN
2010-01-05 NaN
2010-01-06 NaN
2010-01-07 NaN
2010-01-08 NaN
2010-01-11 NaN
2010-01-12 NaN
2010-01-13 NaN
2010-01-14 NaN
2010-01-15 24.041
2010-01-19 24.053
2010-01-20 24.024
2010-01-21 23.965
2010-01-22 23.848
2010-01-25 23.742
2010-01-26 23.682
2010-01-27 23.651
2010-01-28 23.558
2010-01-29 23.340
2010-02-01 23.148
Name: Close, dtype: float64
plt.figure(figsize=(20,3))
plt.plot(gs.Close)
plt.plot(gs.Close.rolling(50).mean())
[<matplotlib.lines.Line2D at 0x7fb9f289bc90>]

plt.figure(figsize=(20,3))
plt.plot(gs.iloc[:400].Close, label="original")
plt.plot(gs.iloc[:400].Close.rolling(50).mean(), label="rolling")
plt.plot(gs.iloc[:400].Close.rolling(50, center=True).mean(), label="center")
plt.legend();

plt.figure(figsize=(20,3))
plt.plot(gs.iloc[:400].Close.rolling(10).mean())
[<matplotlib.lines.Line2D at 0x7fb9f26eff50>]
